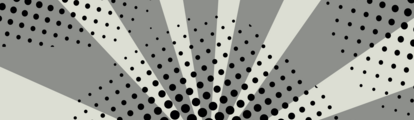
RNA: The Villain… and the Hero
How macromolecules and machine learning can work together to expand the frontiers of precision medicine
Most stories have a hero and a villain – but we don’t often see the same character take on both roles. You may have chosen to forget Superman II (and possibly Superman III), but I am sure COVID-19 is still very fresh in your mind. In this sickening plot, RNA plays a villain – a coronavirus that challenged our immune systems and wreaked havoc around the world. But the best stories have a twist, and RNA has also become a hero – taking the form of mRNA vaccines that allow our immune system to prepare for infection through antibodies offering adaptive immunity. These two vastly different roles showcase a macromolecule whose dynamic nature makes it biologically fascinating.
The next chapter
In fact, our bodies tell a story written by RNA. And the “RNA world” hypothesis suggests that life on earth sprang forth from a simple RNA molecule with the ability to replicate itself – a model that distills life down to the simplest building block possible. The many complex biological processes happening in our bodies at any given time are not only driven by RNA; they can also be quantified by “reading” that RNA. Through this analysis, we’ve begun to understand the pathways and signals involved in health and disease – information we’re now using to build the next generation of medicine, including both treatments and diagnostics.
The boom of RNA data available to study is thanks to advancements in next-generation sequencing (NGS) that have exponentially decreased the cost – and increased the output – of both DNA and RNA data. This technology yields thousands of data points for analysis – but, often, we overly distill these large datasets down to individual signals that are easier to interpret. This approach has limited our scope of understanding when it comes to the complexities of biology. We have learned in recent years that nucleic acid analysis is like watching a play – you can’t focus on just one of the characters, but must observe them all to understand the story. Therefore, the next chapter of RNA – focused on enabling more precise medicine – evolves from measuring a single molecule to modeling many RNA molecules to better represent biological systems. This multidimensional approach has fueled efforts for precision medicine across a number of diseases.
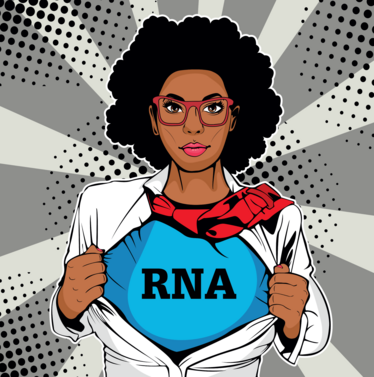
The play’s the thing
One such biological screenplay is in the field of immuno-oncology (IO). Our immune system is modulated by most oncology treatments, including both IO and standard treatments, such as chemotherapy and radiation. It has become commonplace to measure a patient’s immune profile before and after therapy to understand how the immune landscape impacts tumor response. Current efforts to measure this in solid tumors begin by analyzing tumor tissue. In this application, where tissues are formalin-fixed and paraffin-embedded following biopsy, RNA technologies are arguably the best method for analysis. These tissues are unsuitable for flow cytometry and, although multiplex imaging has advanced significantly, there are still technical limitations to the number of signals we can measure. Using RNA sequencing, we can measure even highly degraded transcripts in a multiplexed fashion, allowing us to detect all of the “characters” to more fully understand what’s happening at the site of the solid tumor.
Early approaches using RNA data to measure the immune components of the tumor were influenced by flow cytometry and cell surface markers. They focused on one or two transcript levels as a proxy for immune cells: high CD14 expression = monocytes, high FOXP3 expression = T regulatory cells, and so on. This is not ideal because single markers are not sufficient to uniquely define these immune cells in the tumor microenvironment. Inaccurate measurements led to inconclusive results that did not hold up when moved from the research lab to clinical practice. And immune cell composition is only one facet of an immune response; other RNA signals, including immune escape or co-inhibitory and co-stimulatory signals, also play a role. Essential to building technology that has clinical utility are more robust immune cell measurements, as well as the ability to look at every character in the play.
Comprehensive detection is only the beginning of a successful performance; interpreting the resulting data is a challenge in itself. A cast list cannot tell you what happens in the play. Thankfully, while NGS evolved to generate massive amounts of RNA data, machine-learning technologies expanded in parallel with new approaches suitable for modeling and identifying signals in the data. With these tools, we are now looking at RNA data in new ways to help us characterize and understand disease.
One of the key challenges that machine learning can address is converting RNA signals to immune cell quantification, through a process called “deconvolution.” Various approaches to immune cell deconvolution have been developed and evaluated; a benchmarking study in late 2020 found that the performance relied on a number of factors including data transformation, scaling/normalization strategy, cell profiles or markers, and the deconvolution method itself (1). Not included in this study is a new method for building and deploying multidimensional RNA models that improves upon many of the performance factors described in the paper: immune Health Expression Models, or iHEMs (2). These models are built using machine learning to identify key signals from bulk RNA-seq data generated from purified immune cell populations (see Figure 1). These models enable better signal-to-noise ratios and higher specificity in quantifying immune cells in a heterogenous tumor microenvironment, leading to a more robust understanding of the immune signals modulated in response to disease, therapy, and other environmental factors. What’s more, these models can even be built for nuanced cell states – historically cumbersome or even unachievable with other technologies. The combination of RNA and machine learning in these models bridges RNA sequencing data and immune cell quantification, representing a powerful new tool for IO.
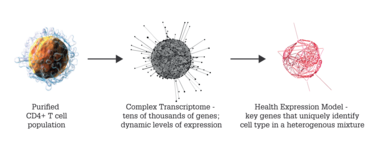
Figure 1. Machine learning is used to build iHEMs by analyzing whole transcriptome data consisting of thousands of signals to simplify into an essential-gene model. Data points in the models represent individual transcripts and their distance from the center represents expression level. T cell image from (3).
A superhero origin story
However, this optimized data simplification does not accomplish the holistic characterization that would provide the most meaningful view of tumor response. To achieve this, we need to upgrade RNA from hero to superhero! We now need to use machine learning to complexify, rather than simplify, our dataset. After measuring these immune cell types, subtypes, and cell states in a clinically annotated cohort of therapy responders and non-responders, we can combine the individual signals into a multidimensional biomarker that better predicts response compared with the individual analytes alone (4,5). This process – predictive immune modeling – evaluates all possible signal combinations to build the most powerful biomarker for predicting disease response. And this heralds a shift in how clinicians use biomarker data. By combining many biological signals into easy-to-interpret prediction tools, we capitalize on our ability to measure massive amounts of dynamic RNA data in a meaningful way.
The COVID-19 pandemic put both RNA and the need for molecular diagnostics front and center in our minds. The world saw firsthand the challenges of building, validating, and deploying a clinical test. As we reflect on the incredible science and collaboration that came out of the pandemic, it’s clear that new diagnostic technologies are an integral part of precision medicine. What’s more, the role of RNA as a powerful tool in our precision medicine toolbox has been solidified. Although we’ve applied this technology to one field of medicine – oncology – its potential applications are vast. With the help of machine learning, RNA is poised to become the superhero in the story of precision medicine.
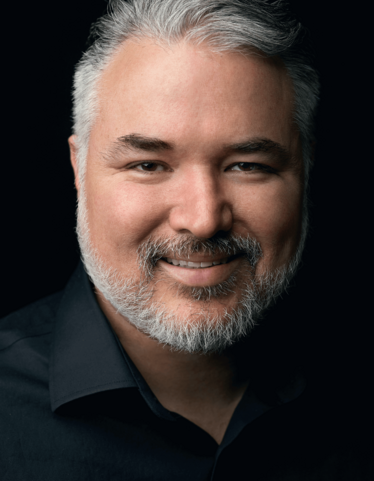
- F Avila Cobos et al., “Benchmarking of cell type deconvolution pipelines for transcriptomics data,” Nat Commun, 11, 5650 (2020). PMID: 33159064.
- I Schillebeeckx et al., “Analytical performance of an immunoprofiling assay based on RNA models,” J Mol Diagn, 22, 555 (2020). PMID: 32036085.
- Blausen Medical, “WikiJournal of Medicine: Medical gallery of Blausen Medical 2014” (2014). Available at: https://bit.ly/3g623kF.
- D Adkins et al., “A multidimensional gene expression model that accurately predicts tumor response to pembrolizumab or nivolumab,” Int J Radiat Oncol Biol Phys, 106, 1132 (2020).
- NE James et al., “Immune modeling analysis reveals immunologic signatures associated with improved outcomes in high grade serous ovarian cancer,” Front Oncol, 11, 622182 (2021). PMID: 33747935.
Founder and CEO of Cofactor Genomics, San Francisco, California, USA.