Making Spatial Biology Actionable
How could spatial biology help patients who don’t respond to immunotherapy?
Ori Zelichov | | 4 min read | Opinion
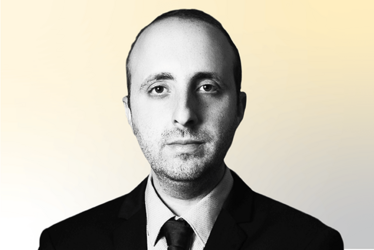
Over the last decade, the field of cancer therapy has undergone a revolution, thanks to the emergence of immune checkpoint inhibitors. But though these therapies provide durable clinical benefit for several cancer types, some of the hype has since faded due to a lack of response in many patients.
Many factors influence response to treatment, but we now know that the success of immunotherapy is highly dependent on the state of the tumor microenvironment (TME) – the complex ecosystem of cells that surround and interact with the tumor. Broadly speaking, the TME can divide tumors into “hot” and “cold” varieties characterized by a high or low abundance of immune cells in the TME, respectively.
This separation holds an essential predictive and prognostic value because “hot” tumors respond to immunotherapy in multiple types of cancers, whereas “cold” tumors are mostly resistant to these agents. Due to its importance in cancer biology, the TME has become part of Hanahan and Weinberg’s revamped “hallmarks of cancer” list (1,2).
The TME’s important role in predicting immunotherapy response led many scientists to rigorously analyze it for novel markers to help identify potential responders. Although proteins that protect tumors from immune elimination, such as PD-1 and PD-L1, are correlated with immunotherapy response, they lack the specificity and sensitivity required to accurately predict response. Today, efforts are being made to develop new technologies to capture the TME more extensively (3). These include new omics analysis methods for genomic and transcriptomic data, improved imaging tools, and multiplex staining. Because the TME encompasses many cell types and cell-cell interactions, in-depth analysis using these advanced tools will unquestionably reveal better marker-based prediction tools for immunotherapy.
Because TME information is spatial by nature, one of the most promising ways to capture the TME’s complexity and its role in drug response is by analyzing biopsy slides. Computer vision algorithms could extract millions of data points from whole-slide images to provide a comprehensive description of the tumor and TME and identify the subtle immune signatures that distinguish between responders and non-responders (4,5). Identifying immunotherapy responders could help improve treatment selection in clinical trials and in the real world, but it doesn’t solve the greatest problem – patients who are not good candidates for immunotherapy and therefore lack effective treatment options. This raises an important question – can we harness the power of spatial biology and artificial intelligence (AI) to not only analyze the TME in a passive way to classify patients, but also find ways to reprogram it from a “cold” state into a “hot” one?
In recent years, new drugs have been proposed to do just that. Reprogramming aspects of the immune compartment – such as immunosuppressive cells – may overcome resistance mechanisms in the TME and enhance antitumor immunity (6). Because the TME is more penetrable and accessible than tumor cells, therapeutic strategies targeting it are appealing. These include drugs that work on myeloid cells, stromal cells, and other types of cells that control hypoxia, angiogenesis, and immune response. The most prominent approach to date is the combination of VEGF inhibition and immunotherapy. VEGF inhibitors have been shown to modulate the TME indirectly (by enhancing tumor perfusion in the TME) and directly (by inhibiting immunosuppressive cells). Recently, a phase III clinical trial revealed that combining chemotherapy with immunotherapy (atezolizumab) and VEGF inhibition (bevacizumab) significantly improved progression-free and overall survival among patients with metastatic non-squamous non-small cell lung cancer (7). These early successes will lead to many other clinical trials combining immunotherapies with TME-targeting agents.
But, to turn knowledge regarding “hot” and “cold” spatial arrangements into actionable treatment decisions, we need to know not only what the TMEs of responders and non-responders look like, but also the expected changes in cell spatial distribution and tissue morphology upon administration of a certain drug. And to do this, we need comprehensive analyses of pre- and post-treatment biopsies to identify the cells and protein interactions required for anti-tumor immunity. Though the emergence of new high-plex spatial proteomic technologies are getting us closer to grasping the complexity of the TME from a spatial perspective, they are still extremely costly and demand months, if not years, of work to analyze the data before concrete conclusions can be drawn. Given the complexity of these high-plex images and the massive amounts of data generated by analysis, AI-powered technological tools will be needed to accurately measure all the different parameters across multiple heterogeneous tumors and make insights from the TME actionable.
The emergence of new drugs that target the TME could potentially expand immuno-oncology’s ability to treat unaddressed patient populations with unresponsive tumors. Using advanced AI tools for in-depth tissue analysis could become a leading way to learn more about the TME, understand drug mechanisms of action, and guide the discovery and development of a new class of immuno-oncology drugs.
- D Hanahan, R Weinberg, “Hallmarks of cancer: the next generation,” Cell, 144, 646 (2011). PMID: 21376230.
- D Hanahan, “Hallmarks of cancer: new dimensions,” Cancer Discov, 12, 31 (2022). PMID: 35022204.
- A Bagaev et al., “Conserved pan-cancer microenvironment subtypes predict response to immunotherapy,” Cancer Cell, 39, 845 (2021). PMID: 34019806.
- E Ofek et al., “Predicting response to pembrolizumab in non-small cell lung cancer, by analyzing the spatial arrangement of tumor infiltrating lymphocytes using deep learning,” J Clin Oncol, 39, 9045 (2021).
- GT Gibney et al., “Predictive biomarkers for checkpoint inhibitor-based immunotherapy,” Lancet Oncol, 17, e542 (2016). PMID: 27924752.
- M Datta et al., “Reprogramming the tumor microenvironment to improve immunotherapy: emerging strategies and combination therapies,” Am Soc Clin Oncol Educ Book, 39, 165 (2019). PMID: 31099649.
- MA Socinski et al., “Atezolizumab for first-line treatment of metastatic nonsquamous NSCLC,” N Engl J Med, 378, 2288 (2018). PMID: 29863955.
Vice President of Clinical Development at Nucleai, Tel Aviv, Israel.