Label-Free Virtual Staining and Cancer Classification
How a deep learning method automates histology imaging processes, achieving 98 percent accuracy in cancer diagnosis – without traditional dyes
Jessica Allerton | | News
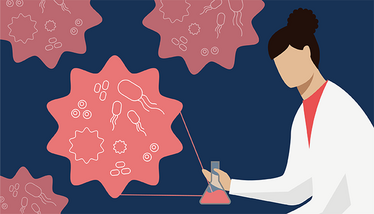
Researchers have developed a new deep learning-based framework that enables virtual staining, segmentation, and classification of histological images without the need for traditional dyes. The approach, centered around photoacoustic histology (PAH), offers a label-free alternative to standard hematoxylin and eosin (H&E) staining – a critical technique for pathological diagnostics but hindered by its labor-intensive processes.
The study integrates three deep learning methodologies into a unified system. First, the team introduces the Explainable Contrastive Unpaired Translation method, which virtually stains grayscale PAH images, transforming them into near-perfect replicas of H&E-stained images. This model preserves critical morphological details, such as cell nuclei and cytoplasm, enhancing the interpretability and traceability of the stained images.
Next, in the segmentation step, a U-Net architecture model segments features, such as cell area, cell count, and intercellular distance within the stained images. These features are essential in distinguishing between noncancerous and cancerous tissues – providing a quantitative foundation for further classification. The segmentation achieved significant accuracy in this study; however, slight discrepancies in cell area measurements were noted in cancerous tissues due to the limitations of PAH images.
Finally, the framework’s Stepwise Feature Fusion method combines features extracted from PAH, virtually stained H&E, and segmented images to achieve a classification accuracy of 98 percent. This outperformed conventional PAH classification, which had a 95 percent accuracy. The model’s sensitivity reached 100 percent, as verified by three pathologists, underscoring its potential for use in clinical settings.
This interconnected deep learning framework, capable of performing automated virtual staining, segmentation, and classification, offers a promising solution for digital pathology. Its high accuracy and automated nature could significantly reduce the time and labor involved in histopathological diagnostics – potentially improving intraoperative decision-making processes.
Deputy Editor, The Pathologist