
History's Mysteries Unlocked
Using animal population models and artificial intelligence to understand tuberculosis
Thomas Tavolara, Muhammad Khalid Khan Niazi, Thomas Westerling-Bui, Metin Gurcan, Gillian Beamer |
At a Glance
Tuberculosis has evolved alongside humans and animals for thousands of years. Although it sounds archaic, the disease still kills over a million people each year. We need a full understanding of Mycobacterium tuberculosis, and, to do that, we must study diverse populations – not just genetically identical individuals.
Tuberculosis (TB) may sound like a disease of the past, but this is a grave misconception. Although TB is not common in the developed world, it causes more deaths than HIV/AIDS or malaria, worldwide. Further, antibiotic resistance has increased, making it harder to treat some TB patients. TB is caused by a fascinating bacterium; Mycobacterium tuberculosis. Over thousands of years, M. tuberculosis adapted to life in the human body, developing abilities to persist asymptomatically in resistant hosts and cause lung-damaging inflammation in susceptible hosts. This inspires our research question: What controls host susceptibility? Nine out of 10 people infected with M. tuberculosis do not become ill. They don’t show symptoms, they don’t need treatment, and they aren’t contagious. What are the differences between the majority who never become ill, and the small fraction who do develop active TB? We seek explanations for these differences from the perspective of host genetics. We hypothesize that genetic differences in hostscan help explain differences in susceptibility to M. tuberculosis. More specifically, we think increased susceptibility reflects genes that increase inflammatory signaling when cells detect M. tuberculosis bacteria in the lungs.
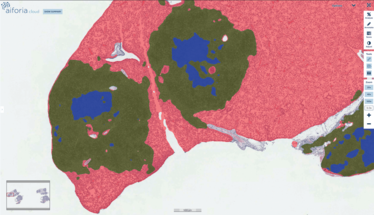
A model population
To address our hypotheses, we use the Diversity Outbred mouse population. The DO population has as much genetic diversity as the human population and like individual people, each DO mouse is genetically unique. We are modeling (to the best of our ability), the genetic diversity of the human population and the range of responses that humans exhibit when infected with M. tuberculosis.
Experimentally, we can identify the genetic makeup of DO mice who are susceptible (or resistant) to M. tuberculosis. By studying how DO mice respond to M. tuberculosis, we can locate regions of the genome (i.e., specific DNA segments) that are associated with responses to M. tuberculosis. This is a mechanistic approach to understanding TB. We are also using information from DO mice to build artificial intelligence (AI) models that can classify (diagnose) and predict (prognose) outcomes to infection. First, we identify the features that correlate with susceptibility or resistance to M. tuberculosis in the DO studies. Then, we test whether the features can accurately diagnose or prognosticate the host outcome in a blinded fashion on new experimental data. We are trying these approaches with many data types: genomic, gene expression, protein biomarkers, capacity to restrict M. tuberculosis growth, etc. Now, we are applying AI to lung histology – granulomas, cellular and tissues responses – any visual manifestation of the host response.
Our work
We found the DO mouse population response to M. tuberculosis similar to that of humans. Some individuals are very susceptible – becoming ill within a few weeks – whereas others are quite resistant and appear healthy for months or even years. These widely different outcomes occur in adult mice of the same age given the same amount of bacteria at the same time on the same day. All mice live in the same environment. The one thing different about each mouse is its genetic background – so the different responses can be explained by different sets of genes that control the host’s response to M. tuberculosis. Using this mouse population, we want to identify the genes and pathways that lead some hosts to develop early and severe lung-damaging inflammation. If we can identify a cause of lung-damaging inflammation, we may be able to target a pathway for treatment in patients with active tuberculosis. We also found that certain combinations of molecular biomarkers better discriminate which DO mice are susceptible to pulmonary tuberculosis than single biomarkers. This too links to some underlying genetic phenomenon we hope to trace and exploit so that we can identify which mice will be susceptible to M. tuberculosis infection.
Many genes are involved in a host’s overall resistance or susceptibility to M. tuberculosis. We think we are discovering that lung-damaging inflammation and M. tuberculosis restriction are controlled by different pathways. I am surprised by that preliminary conclusion, because I had previously imagined that infection and inflammation were somewhat of a chicken-or-egg situation – nearly impossible to tease apart. With the DO mouse population, we can start to differentiate between the host pathways that cause lung-damaging inflammation and the host immune responses that restrict bacteria. From an AI perspective, we may also conclude that the degree of density and distribution of nuclear debris in lung tissue may be a predictive factor for susceptibility.
In the future, we plan to undertake detailed studies of the specific genes and pathways that cause TB in susceptible individuals. A greater understanding of disease will lead to better individual risk assessment and prognosis, as well as improved vaccination strategies, therapies, and monitoring. We will also use AI to tease apart specific imaging biomarkers indicative of different disease susceptibilities. We may be able to map between different information domains, predicting pathology from gene expression data or simulating tissue given a specific mouse genotype. Through these studies, we will gain a much better understanding of the relationship between genotype and phenotype.
We are also using the DO mouse population to identify and validate biomarker signatures that can predict disease outcome before infection occurs. We may have a translational advantage because we are working with a genetically diverse population – which is why I’m so grateful to the investigators who had the vision and capacity to push the development of the DO mouse population. Their service to science is immense.
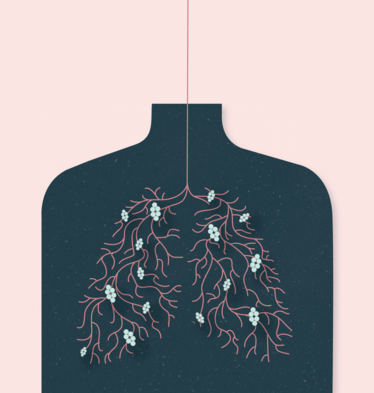
A helping hand from AI
Most pathologists recognize patterns of microscopic change (lesions); group the lesions into clinically relevant states (diagnosis); attempt to predict host outcomes (prognosticate); and integrate clinical or research data in context to understand how disease occurs. AI can help pathologists perform the first three tasks consistently and efficiently. The premise of the latest AI trends is that given enough examples of a feature, such as a granuloma in a lung section, AI can also accurately classify that feature. Further, an AI system can also learn to reliably classify the disease state of a patient given enough prior examples of that disease in other patients. Like pathologists, AI can use many different data sources – hematoxylin and eosin-stained sections to clinical laboratory data and constitutional symptoms. The concept of mapping a domain of information (imaging or otherwise) to a specific outcome is called classification. When a pathologist performs this process, it’s called diagnosis.
A (brief) overview of AI in tuberculosis
We are not the first group to use AI tools for TB research. Many publications demonstrate methods to detect M. tuberculosis bacilli in digital images of sputum samples. These methods generally follow two basic steps – segmentation of M. tuberculosis bacilli and classification of segmented objects as bacilli and non-bacilli.
Segmentation generates potential candidates for bacilli by examining the color of the pixels in the image. In lung tissue sections from M. tuberculosis infected DO mice, acid-fast staining colors the bacteria red and the rest of the tissue has a blue counterstain. Thus, red pixels are simply classified as part of bacilli, and every other pixel is classified as background. Specific methods for partitioning red pixels from the background include thresholding, clustering, artificial neural network approaches, Bayesian segmentation, and fuzzy segmentation. Once potential bacilli candidates are generated via segmentation, they are filtered for false positives (due to small artifacts in the image) through feature extraction and subsequent classification. Features extracted from the bacilli candidates include perimeter, eccentricity, area, Fourier descriptors, and Hu’s moments. Classifiers on these extracted features include support vector machines, Bayesian classifiers, and artificial neural networks.
In addition to detecting bacteria, AI tools may be used to diagnose TB in chest radiographs or other types of patient scans. These methods generally use deep learning to classify radiographs or scans as active pulmonary TB or healthy. This classification is sometimes preceded by a segmentation of cavities characteristic of severe lung damage. These AI methods require a ground truth segmentation prepared by a radiologist.
Though these methods are accurate in diagnosing active pulmonary TB, they don’t predict who will develop the disease, or to what degree. We envision results from studies in M. tuberculosis infected DO mice may translate to a better ability to identify who is susceptible to TB, when they will develop TB, and the severity of disease. To this, we need AI tools to turn the visual information from the granulomas of M. tuberculosis-infected DO mice into quantifiable data suited for statistical analyses and machine learning. In essence: AI to detect, localize, classify, and quantify visual patterns into actionable information.
How we use AI
In pursuit of these goals, we use AI in several projects to automate mundane or time-consuming tasks, model complex imaging data, and discover underlying phenomena in histopathology images. We use AI to automatically detect and quantify features of interest within lung granulomas. That can straightforward such as counting macrophages, which is easy for one granuloma, but not feasible to scale up. It’s difficult for a pathologist to crank through 1,000 slides. High-throughput quantification is easy with AI but challenging for your pathologist… unless you are trying to get them to quit.
As an example, we’ve made an algorithm, using the Aiforia platform, that can count foamy macrophages within granulomas. Hundreds or even thousands may be present in a single granuloma. It’s impossible for a person to count thousands of macrophages in thousands of sections from over 1,000 mice. I wouldn’t eat or sleep; I would be chained to my microscope (and my thumb would hurt from pressing my counter so many times). I’ve turned to AI to extract foamy macrophage numbers that we use in downstream analyses. AI spared me a tedious task and saved my thumb.
Beyond granulomas, we’ve developed AI tools to automatically segment necrosis, lymphocytic cuffs, macrophage-rich regions, neutrophil-rich regions, infected tissue, and healthy tissue in hematoxylin and eosin-stained lung sections. In these tasks, we are interested in geographical relationships between the location and distribution of different cell types, and anatomical structures that may indicate susceptibility to TB. And though we already have robust method to localize all nucleated cells (H&E) and to segment M. tuberculosis bacilli (acid-fast), there’s no way I could enumerate all those relationships. So instead, AI can automatically identify those anatomic sites, localize cells or bacilli, compute complex relationships between and among these regions and their cells, and turn that into something useful, such as predicting the susceptibility of that DO mouse.
Recently, we developed an exciting AI tool (which I refer to as our “bag of tricks”) that automatically identifies imaging biomarkers for susceptibility using only lung histopathology images and the susceptibility category assigned to each DO mouse. More specifically, we have focused on the “supersusceptible” DO mice that develop pulmonary TB within eight weeks of infection. Usually, AI tools require that pathologists manually annotate diseased areas on a slide to provide a ground truth. However, here we developed an AI tool to diagnose supersusceptible DO mice using only the category label and the lung histology image. No manual annotation was needed. Further, what was incredible with our “bag of tricks” method is that the AI automatically extracted a feature clearly interpretable by any pathologist. In this case, the feature AI used to classify supersusceptible DO mice – which we are dubbing an “imaging biomarker” – corresponded to pyknotic nuclear debris. This is useful for our TB research, but also has broad implications for the field of computational pathology, in which diagnosis labels at the slide level can yield interpretable imaging biomarkers, discover new image features of diseases, and help to validate existing clinical biomarkers.
Now that we can comfortably delegate to AI, what should pathologists do? Our brains are our best asset. Our most important contribution to research and patient care is thinking – integrating information, solving complex problems, and asking the next set of questions. What do the observed changes mean in biological context? What did we learn about the pathogenesis of disease? Is this a new disease? That’s what I want our jobs to be.
Doctoral Candidate, Wake Forest University, Winston-Salem, North Carolina, USA.
Assistant Professor, Wake Forest School of Medicine, Winston-Salem, North Carolina, USA.
Senior Scientist at Aiforia Inc, Boston, USA.
Director of the Clinical Image Analysis Laboratory, Wake Forest School of Medicine, Winston-Salem, North Carolina, USA.
Assistant Professor, Department of Infectious Disease and Global Health, Tufts University’s Cummings School of Veterinary Medicine, North Grafton, Massachusetts, USA.