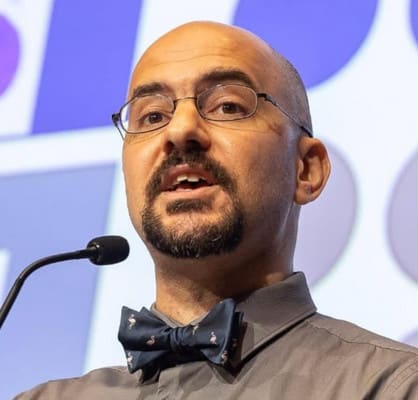
Andrew Janowczyk
Assistant Professor, Department of Biomedical Engineering, Emory University and Georgia Institute of Technology, Atlanta, Georgia, USA; Department of Oncology, Division of Precision Oncology, Department of Diagnostics, Division of Clinical Pathology, Geneva University Hospitals, Switzerland