What’s New in Digital Pathology
The research and developments that caught our attention – from AI lung cancer classification to open source microscopy image analysis
Jessica Allerton | | 3 min read | News
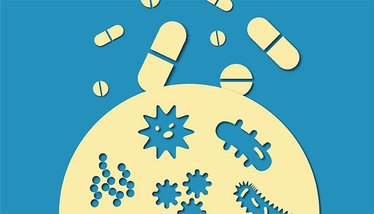
Twice the power
Lung adenocarcinoma (LUAD) accounts for 40 percent of lung cancer cases, but the current gold-standard diagnostic process can be labor intensive and time-consuming. In response, a team of Chinese researchers propose a “weakly supervised learning” method for LUAD classification on label-free tissue slices with virtually stained histology samples (PMID: 38588884). This method provides a cost-effective and rapid approach to postoperative examinations. Moving forward, other label-free imaging modalities and disease contexts could be developed using this method as a blueprint.
Onco-algorithm
A new AI-powered whole-slide pathology foundation model could set a new benchmark in digital pathology. The Providence Gigapixel Pathology Model (Prov-GigaPath) uses large-scale real-world data and novel vision transformer architecture to complete various digital pathology tasks (PMID: 38778098). It was designed with hopes of tackling the scarcity of publicly available pathology data and a resounding difficulty in designing model architecture for capturing global and local patterns across whole slides. This technology demonstrates significant advancements in mutation prediction, cancer subtyping tasks, and vision-language processing. Prov-GigaPath shows potential for enhancing clinical diagnostics and also suggests applicability to broader biomedical domains for self-supervised learning from high-resolution images.
Undesirable clumping
Protein clumping is the root of a wide range of neurodegenerative disorders, including Alzheimer’s disease. To learn more about its characteristics, researchers at the University of Copenhagen have developed a machine learning algorithm to track clumping proteins in real time (PMID: 38409214). The SEMORE (SEgmentation and MORphological fingErprinting) algorithm automatically maps and tracks important characteristics of clumped proteins down to the nanometer scale in microscopy images. SEMORE also counts and groups clumps according to their shape and size while continuously tracking their development, which can have a major impact on function and behavior. Future developments of SEMORE could support the development of new neurodegenerative disorder drugs and therapies.
AMR mapping
A new study uses AI to uncover gaps in global antimicrobial resistance (AMR) research (PMID: 38723455). After compiling a comprehensive database of 254,738 articles spanning two decades, the team discovered significant inconsistencies in semantics and methodologies. Through their sophisticated AI-based analysis, the team developed global maps highlighting where the burden of increasing AMR is most acute. These researchers call for a greater harmonization of research methods, suggesting that culture-based and genomic AMR analysis is crucial for both data integration and holistic health solutions across the globe.
Brain tumor subtyping
Researchers create a new AI tool for quick and accurate classification of brain tumors – opening avenues for central nervous system tumor diagnosis in clinics (PMID: 38760587).
AI immunophenotyping
Machine-learning-based CD8 immunophenotyping models identify patients with non-small cell lung cancer that could benefit from immunotherapy (https://doi.org/10.1089/aipo.2023.0008).
Open source analysis
DL4MicEverywhere – an open source platform for microscopy image analysis – launches to address challenges in computing resources for life sciences research (PMID: 38760611).
Mutating data
Machine learning methods offer rapid and effective diagnosis of mutations in gliomas, but inconsistent standards remain a barrier in clinical applications (PMID: 38539436).
Deputy Editor, The Pathologist