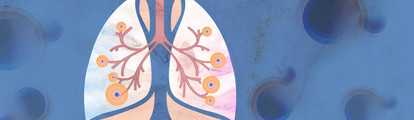
Watch This Space!
Could the spatial arrangement of immune cells help reveal when aggressive chemotherapy is the right course of action for early-stage lung cancer patients?
At a Glance
- The number of tumor-infiltrating lymphocytes (TILs) is associated with patient outcome in early stage lung cancer
- A new automated method uses the spatial arrangement of TILs to predict which early stage patients will benefit most from chemotherapy
- The prognostic signature separates patients by risk of disease recurrence and uses a deep learning approach to analyze digital pathology images
- Predicting the chance of disease recurrence is vital; up to 55 percent of patients relapse within the first five years
Patients with early-stage lung cancer have a head start from a diagnostic point of view. But once their disease has a name, it’s important to press that advantage by giving them the right treatment as soon as possible. However one of the critical problems in early-stage lung cancer is predicting whether or not patients will benefit from aggressive chemotherapy. In other cancers, predictive assays allow clinicians to identify which patients will receive added benefit of more aggressive chemotherapy; unfortunately, there are no such tests for lung cancer – the most fatal cancer worldwide. My colleagues and I recently discovered that the arrangement of tumor-infiltrating lymphocytes (TILs) is a prognostic signature of early recurrence in early-stage lung cancer. Subsequently, we developed an automated technique that uses their spatial architecture to predict patient outcomes.
Looking into space
Much of my career has involved linking computer-aided diagnostics with digital pathology, including the application of methodologies and algorithms for the analysis of digital pathology slide images. Over the last 8–9 years, our focus has shifted a little; we wanted to move away from using computational pathology as a form of decision support for the pathologist, and instead explore how computational pathology could play a direct role for the clinician. More specifically, we wanted to help oncologists modulate therapy and manage patient treatment. At the moment, we are attempting to use computational algorithms in conjunction with digital pathology images to find patterns that inform us about the aggressiveness of disease. We hope that these will ultimately help us predict potential responses to therapy. In this respect, our goal has evolved from merely supporting the pathologist to striving to develop companion diagnostic tools for disease risk stratification and treatment response assessment, making decisions easier for the oncologist.
I’ve been particularly interested in the concept of spatial nuclear architecture for a long time, especially in the context of grading breast and prostate cancer. The idea really took off when we started looking not only at the shape and number of immune cells, but also at how their spatial arrangement and architecture could be used to improve the grading of breast and prostate cancer, and therefore benefit the pathologist. It’s already well known in lung cancer that TIL number plays an important role in determining prognosis and treatment response. But, because there is a subjective element to the manual counting of TILs, the challenge has always been trying to address issues with inter-reader variability. For that reason, the first ambition of our project was to use the spatial architecture of TILs to aid pathologists in counting. In addition, we also wanted to use the spatial arrangement of features to forecast prognosis and disease recurrence in early-stage lung cancer patients.
It turned out that spatial arrangement was extremely important; in fact, significantly more so than the manual enumeration of TILs. These features clearly separate the patients who are at high risk of disease recurrence (and who would therefore likely benefit from adjuvant chemotherapy following surgery) from those who are at a lower risk of recurrence and may not need adjuvant chemotherapy. The spatial architecture of TILs was prognostic in every one of our data sets, providing good validation of our initial hypothesis that arrangement and architecture are critical.
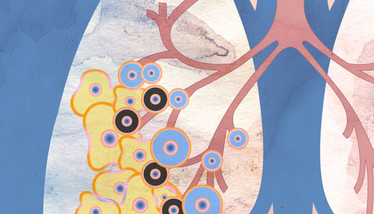
Dipping into deep learning
At the moment, deep learning is the go-to technology for many aspects of digital pathology. We used targeted deep learning to find the TILs and cancer nuclei initially, but the subsequent spatial features and architecture were captured using a non-deep learning methodology known as a handcrafted feature approach (which we adopted to imbue some domain knowledge into the process). One of the most critical aspects to me as a bioengineer is that there has to be feature and model interpretability; there has to be some degree of intuition built into the classifier. That’s why, for me, the most satisfying part of this research is that it resonates with something we already know about morphology of the disease: that TILs are important.
I believe this combination of transparency and intuition is going to be a critical facet of clinical adoption. One of the challenges with deep learning is that, sometimes, it doesn’t quite provide that transparency, and I have my concerns that a lack of intuition and interpretability could potentially impede clinical adoption. Before clinicians make a call as to whether or not chemotherapy is needed, they are going to want to know exactly what’s “under the hood.” And that’s why I find our approach so satisfying – because it resonates with what we know about the domain and has intuitive appeal.
A vital aspect of the success of our test lies in making it simple for the end user – the oncologist. In essence, we’re providing a risk score that gives the oncologist easy access to clinically actionable information, so we want to minimize the need for training and learning.
Space for progression
We’ve already established that we have a prognostic signature, and we believe that it will be validated in the future as predictive of the potential added benefit of adjuvant chemotherapy. We are striving to begin clinical trials that will look at patients previously treated with surgery or surgery plus chemotherapy. Of those who were treated with surgery, we want to show that we can identify which patients had a higher risk of recurrence and would have benefited from adjuvant chemotherapy. Additionally, of the patients treated with surgery plus chemotherapy, we will attempt to identify the low-risk patients who may not have needed chemotherapy.
I think the signature also carries significant implications for other therapeutic modalities. There is currently a great deal of excitement surrounding immunotherapy, and there has been much interest in trying to understand whether patients who have more TILs will exhibit a better potential response to immunotherapy than those with fewer TILs. We have managed to show that the TIL spatial arrangement signature from diagnostic biopsy images also predicted how well patients would respond to immunotherapy. Taken together, these results suggest that our prognostic signature could help identify not only patients who stand to benefit from adjuvant chemotherapy, but also patients who stand to benefit from checkpoint inhibitor immunotherapy for lung cancer.
In terms of a timeframe for our vision, I believe we have a good shot at deploying in clinics within 18–24 months. Once we have managed to prove that our prognostic signature is predictive, we must obtain regulatory approval from the US FDA. The beauty of our approach lies in the fact that we’re not destroying any tissue because we only use digital slides, so our validation studies shouldn’t be too time-consuming. Indeed, we believe the technology can be approved via a somewhat less onerous regulatory pathway.
Going global
The fact that our concept potentially spans multiple different disease indications is very appealing. Joel Saltz and his group from Stony Brook recently wrote an excellent paper that looked at the spatial location of TILs within the tumor and stroma, and found that TIL position was prognostic across multiple types of cancer. In cancers such as ovarian cancer and triple negative breast cancer, the role of TILs has been linked with disease outcome and prognosis. Therefore, although we’ve developed the signature using lung cancer, we absolutely intend to apply and validate it across multiple disease stages and sites. For me, the most exciting part of this research is that it demonstrates the possibility for finding sub-visual signals and patterns in routinely acquired hematoxylin and eosin (H&E) stain slides, which could help guide pathologists and oncologists in the future.
Although we’re working in a relatively new area of research, the impact that digital and computational pathology could have on global health is huge – and that’s very exciting. Expensive molecular companion diagnostic tests have a big market in Europe and North America, but large parts of the world are simply unable to afford these tests. Consider that, because our computational pathology approach can be carried out from an image alone, analysis can be performed for anyone with a specific cancer anywhere in the world. The local pathologist or oncologist would simply upload an image and analyze it with the algorithm to render a prediction – an immediate change to the way cancers are diagnosed, staged, and treated throughout the world.
- G Corredor et al., “Spatial architecture and arrangement of tumor-infiltrating lymphocytes for predicting likelihood of recurrence in early-stage non-small cell lung cancer”, Clin Cancer Res, [Epub ahead of print] (2018). PMID: 30201760.
- X Wang et al., “Interaction of Tumor Infiltrating Lymphocytes and Cancer Nuclei Predicts Response to Nivolumab in Non-Small Cell Lung Cancer (NSCLC)”. Presented at the International Association for the Study of Lung Cancer’s (IASLC) 19th World Conference; September 23-26, 2018; Toronto, Canada.
Anant Madabhushi is the F. Alex Nason Professor II of Biomedical Engineering at Case Western Reserve University, and Director of the University’s Center for Computational Imaging and Personalized Diagnostics, Cleveland, USA.