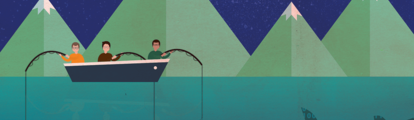
Three Gurus of Big Data
Big data. Everyone’s talking about it, but what exactly is it? How can it be harnessed to advance medical research? And what perils lie within the oceans of data that now surround us? Three experts from different backgrounds go fishing for answers.
At a Glance
- Data collection is easier than ever – but with such high volumes at our fingertips, it can be difficult to ensure we’re using it well
- Before accessing available data, it’s important to understand what was collected and how, to ensure that it’s fit for your purpose
- New kinds of data require new governance, new standards, and new interfaces in order to ensure good quality and comprehensibility
- Big data will be increasingly important for a wide range of applications – but only if our technologies, and our methods, keep up
Gone are the days of trekking to a library to thumb through research papers or handing out paper questionnaires to collect patient data. Now, we can gather terabytes of data at the click of a button. But are we making the best use of the data we’re collecting? Here, data experts Dipak Kalra, Iain Buchan, and Norman Paton join the debate.
Dipak Kalra
Dipak is President of the European Institute for Health Records (EuroRec), and Professor of Health Informatics at University College London, in the UK. As a physician working in London in the early 1990s, he found that the computer systems of the time couldn’t give him the insight he needed into his patients’ data. He joined a European research project on health records and soon realized that creating truly useful electronic health records was a massive and exciting challenge. Twenty-five years on, Dipak is still working to improve health informatics. He also leads a non-profit institute that aims to promote best practice regarding health data in research and communicate with the public about how their health data is used.
Iain Buchan
Iain is Director at the Farr Institute of Health Informatics Research, and Professor in Public Health Informatics at the University of Manchester in the UK. As a medical student, Iain Buchan saw the rise of the PC revolution. It was obvious to him that there was a need to fuse pathophysiological and biological reasoning with a statistician’s view of analysis and inference. Buchan created a statistical software package (www.statsdirect.com) that quickly attracted tens of thousands of users. Over the years, he became increasingly interested in the interplay between medicine, statistics, and public health data. Buchan’s team (www.herc.ac.uk) is addressing what they see as a fundamental flaw in observational medical research – currently, research orbits around data sources, but it should orbit around questions and problems, pulling in data from various sources as necessary.
Norman Paton
Norman is Professor in the School of Computer Science at the University of Manchester in the UK, where he co-leads the Information Management Group. He is a computer scientist by training, with a PhD in object-oriented database systems. His work focuses on data integration, which involves bringing together data from multiple sources in a manner that allows for easy interpretation. Previously, the process has been quite slow and small scale. With the rise of big data, the process needs to be streamlined and made more effective. Paton is currently working in data wrangling – collecting and cleaning up data so that it can be analyzed in an integrated form. Data wrangling is an expensive and time-consuming process, so Paton is working to automate as much of the process as possible.
What is big data?
Dipak Kalra: This is an interesting question, and one that the healthcare community as a whole has yet to conclusively answer. For me, the characteristics of greatest importance are: a large number (millions) of patients, combining multiple data sources (with various interoperability and linkage challenges), and data recorded over time to allow trajectories to be determined. I’m interested to see what answers my colleagues will give.
Norman Paton: I think of big data as more of an era than a specific size or type of data. More and more data is being accumulated from different places, and that creates an opportunity for people to use and exploit it. “Big data” has been used as a blanket term to cover numerous cases in different contexts, so it’s difficult to find a single definition. However, I believe that it reflects a combination of an increasing number of data sources, an increasing number of domains that have a surplus of data, and the variety that exists within those.
Iain Buchan: There are many possible definitions based around the “four Vs” – volume, velocity, variety and veracity – but ultimately, I define big data as big enough to address the challenge at hand – with sufficient accuracy and timeliness to inform better actions.
What impact is big data having on biomedical research?
DK: Big data allows us to finally have fine-grain, routinely collected clinical data. Soon we will be able to look at large numbers of patients retrospectively and at a much lower cost, which will explode our understanding of diseases, treatments, biomarkers, health service care, pathway patterns, and how to optimize patient outcomes. I cannot imagine a more exciting time than this.
NP: Big data allows more diversity in research opportunities. For example, we might want to better understand the efficacy of a certain cancer treatment; every hospital has records, but pooling together the relevant data from all of them would be an unmanageable task. Computer systems need to be developed that make the process of identifying, integrating, and interpreting diverse data sets more cost-effective. In medical sciences, opportunities are everywhere because information is constantly being produced in hospitals, drug trials, labs, and so on. I don’t expect to see one mega project using all the information, but many relatively small-scale, focused projects.
IB: Big data, properly harnessed, gives us bigger science. It allows us to network teams and universities across the world, to collaborate rather than compete. And that collaboration becomes more powerful as the ensemble of data, analytics and experts gets bigger. There are two levels of big data: one is the scale of data and algorithms working machine-to-machine autonomously across locations, and the other is allowing humans to work in a much bigger team. You might think of this as “assisted reasoning for team science.”
How can you ensure the quality of your data?
NP: It’s extremely difficult to gain a clear understanding of your data set. It’s not just a case of “good” or “bad” quality, but knowing whether the data is fit for purpose; what is fit for one purpose may be completely unusable for another. There are many metrics used to measure quality – completeness, accuracy, freshness, and so on, but fitness for purpose may be quite domain-specific.
DK: One has to be careful. When organizations collect data for any purpose (management, tracking, administration, and so on), they select the fields of interest relevant to them and disregard the rest, which is good practice. Then they filter and select the data to fine-tune it further. The problem starts when somebody else wants to use that data for a different purpose, without being aware of all the previous filtering. It creates a risk of misinterpretation.
IB: I think one of the best ways to improve data quality is to “play back” what you have done to the people closest to the data. As soon as you talk to someone familiar with that data supply chain, they are likely to point out problems that might go undiscovered if you just suck up data. This turns tacit knowledge into explicit metadata – increasing the discovery power per unit of data.
What are the greatest challenges when dealing with big data?
DK: I can see four main issues. The first is in establishing trustworthy practices. This era of big data brings a very different set of governance challenges, which require new codes of practice, as well as winning the trust of society and healthcare providers.
The second is interoperability. I think the adoption of standards is too limited, with many data sets and electronic health records applying different internal data architectures and terminology. There needs to be a range of widely adopted standards so organizations and individuals are able to interface with each other and compare data.
The third is data quality, which we’ve already discussed.
The fourth is that, as a field, we have been slow to promote the value we get from health data. When we do make great discoveries from health data, we don’t always make it clear to society or to funders that it was the result of significant investment in IT, as well as helping patients to be more comfortable with how their data are being used.
IB: I would add that a common mistake is naïve translation of tools from one environment to another. I’ve seen cases where dashboards designed for business intelligence have been directly translated into healthcare, which means clinicians are faced with a blizzard of dashboards they don’t have time to digest. When designing user interfaces, we need to take note of basic learning from avionics, where it is long-established that a pilot cannot focus on more than seven or so dials in his or her field of view.
Another common mistake is to apply machine learning to data as if it were an unbiased sample of human health. In medicine, there is so much “missingness” and measurement error in the data, and so many things that can’t be measured directly, that data-structure is meaningless without overlaying prior information about the structure that would be in the data if you could observe it. The mistake is looking for patterns in “buckets” of data when we should be starting with the patterns we know and building more patterns around that. Machine learning requires a very careful approach when dealing with biology and health data.
#datasaveslives
The #datasaveslives social media campaign promotes the positive impact that data is having on health. Projects recently highlighted by the campaign include:
- Connected Health Cities:a project that collates health data from multiple cities and uses advanced technology to analyze it. The goal? To use existing resources to connect disparate services and make patient care better than ever. The three-year pilot project involves setting up a “learning healthcare system” that will continually improve care, as well as identifying care pathways for specific needs.
- SPIRE: an information-sharing system that allows doctors in Scotland to share anonymized, encrypted patient data with researchers. Only information for specific purposes will be collected, and after analysis, the data will be destroyed. What’s the point? To manage patients with long-term conditions, research specific illnesses, and plan future services.
- Streams: a new app that collects patient data and monitors test results. Health care workers can easily access information and request interventions – but best of all, the app features an instant alert system for potential problems. London’s Royal Free Hospital is trialing a version focused on acute kidney injury, and nurses estimate that the app saves them up to two hours every day.
How important is the public perception of big data?
IB: It’s vital. My group has a rule when speaking with those outside the field that we don’t talk about data, databases, or information systems in the first part of the conversation. Instead, we talk about problems that the data can be harnessed to address. We need the public on board to help unravel the vast gaps in our knowledge – for example, how best to treat patients with more than one condition. Take a look at twitter.com/hashtag/datasaveslives to see this in action.
DK: Trust and engagement from the public is mission-critical in the growth of big data and its use in research and healthcare. The public have to be confident that the use of their data is in their interest, and in the long-term interest of society. It’s also important that the patients feel a sense of personal autonomy about health and wellness. To help foster that, I think we should all be able to access and use our own data, to help us make better decisions about our health.
NP: It’s important for the public to have a wider understanding of the opportunities big data presents and how their data is involved with that, but it’s difficult when organizations remain relatively opaque in relation to the use they are making of personal data.
DK: I see a lot of news stories focusing on security breaches or data leaks – a missing CD, a stolen laptop, a USB stick found in a waste bin. It leads to a natural distrust about how organizations look after our data. In reality, most data are very well protected – increasingly so, as we implement state-of-the-art security measures. But we need to increase public confidence.
What are the most common misconceptions about big data?
IB: I think the biggest misconception is that big data is the answer to everything, and that bigger data will always lead to a better answer, which is a myth. Indeed, there are some cases where more raw data can reduce discovery power, when the heterogeneity of the data sources increases but there is no metadata to make that heterogeneity useful in analyses. So, it isn’t a case of getting as much data as possible, but rather finding the most powerful analytics possible with the data. It’s about bigger science, not just bigger data.
NP: I agree that there is too much focus on size. Although big data is often spoken about in terms of the four Vs, it’s easier to get a handle on volume than the others so there is the tendency to associate big data mostly with size.
What are the most important applications for big data?
DK: If I could pick a headline issue for big data to rally behind, it would be that we’re an aging society and the number of patients who have multiple long-term conditions is rising. Our historic scientific knowledge of diseases and treatments have usually been based on the study of single diseases, so our knowledge of how multiple diseases interact is fairly limited. Big data will give us the ability to study populations so that if you have a patient with diseases A, B, and C, and you want to find out the effect of treating them with drug X, you’ll have a sufficient sample size to get a useful answer.
IB: Multi-morbidity is definitely a key priority. As Dipak says, we’re an increasingly aging population with a prevalence of multiple concurrent conditions, and to address that we need actionable analytics – statistical surveillance of primary care and prescription data, with feedback to physicians so they can determine the patient’s best care pathway.
Another area of importance to me is infrequent clinical observation giving way to consumer health technologies that can tap into the rhythms of a patient’s life via wearables or mobile technologies. If I had arthritis, my patterns of movement might reflect a temporal pattern of symptoms currently invisible to the clinic. That takes me to the third area, which is information behavior. If you’re able to use technologies in ways that slot into the rhythms of daily life and don’t annoy people, then they will be used more often and so give a less biased sample. The next step is to help influence health behaviors; for example, getting people to exercise more or to persist with preventative medicines that we might otherwise give up on because of a lack of feedback about the benefits we can’t easily see. Our bodies are our own laboratories in which we run n=1 experiments, which big data and big analytics may bring to life in new ways.
NP: I think big data is going to be important for almost every application. That doesn’t mean it’s going to affect every aspect of everything, but big data is going to crop up everywhere so I personally feel like it’s difficult to narrow down a few specific important applications.
Where do you think the future of big data lies in five years – or 50 years?
DK: There are many exciting prospects for big data over the next five years. Biomarker discovery, using genetic information, metabolomics and proteomics, will become more efficient. Big data could also make assisted technologies more useful for people with functional difficulties. Then, there are sensors and wearables, which are appearing now but will become much more integrated and useful in the future. In the far future, I think the relationship between healthcare professionals and patients will become more symbiotic. Computer applications will be seen less as tools and more as collaborative agents able to provide insight from large volumes of data – almost like a digital colleague or companion.
NP: In the next five years, I think big data processing is going to become more predictable – we will better understand what we can and can’t do with it, and be able to build more mature tools and technologies to support data management and analytics. In 50 years, I believe automation will free up data scientists to focus on how to use data more efficiently, and drive the field forward at a more rapid pace. I don’t think we’re very far from automated software that, for example, can read through scientific papers and extract key information about a particular protein, pathway, or topic you’re interested in. These kinds of applications will make a big difference to a lot of daily life tasks.
IB: Increasingly, we live our lives connected to each other’s behaviors through social digital technologies. In 50 years, I think we’ll be talking a lot more about how we influence health behavior, as individuals and as societies. Therein lies a “big connectedness” of information – the fusion of biology, behavior, and environmental data, and new understanding of how those three principal components interact – that will push healthcare, consumer health, and public health forward as greater than the sum of their parts.
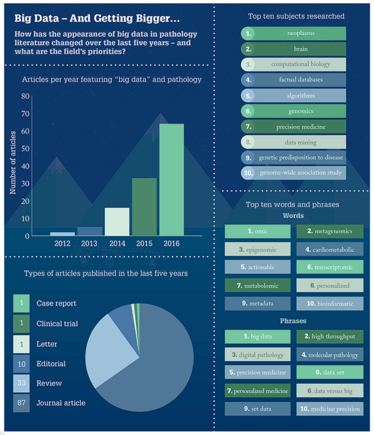
Norman Paton is a Professor in the School of Computer Science at the University of Manchester
Iain Buchan is the Director at the Farr Institute of Health Informatics Research
Dipak Kalra is the President of the European Institute for Health Records