Few topics in modern pathology command as much expectation, excitement – and perhaps fear – as computational pathology. Here, three algorithm advocates debate and discuss the ins and outs of computational pathology – and consider what it means for pathologists now and in the near future…
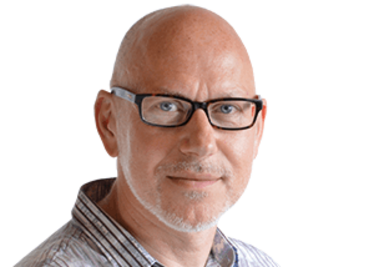
Jeroen van der Laak is Associate Professor in Digital Pathology at the Radboud University Medical Center in Nijmegen, the Netherlands, where he leads a research group focusing on computational pathology. His research investigates the use of deep learning-based analysis of whole slide images for different applications: improvement of routine pathology diagnostics, objective quantification of immunohistochemical markers, and the study of new imaging biomarkers for prognostic use.
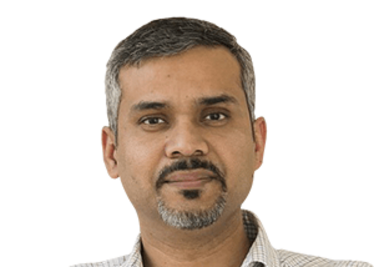
Nasir Rajpoot is Professor in Computer Science at the University of Warwick. He also holds an Honorary Scientist position at the Department of Pathology, University Hospitals Coventry & Warwickshire NHS Trust. He is the founding Head of Tissue Image Analytics lab (formerly known as the BioImage Analysis or BIA lab) at Warwick since 2012. The current focus of research in his lab is on developing algorithms for the analysis of large multi-gigapixel digital pathology images, with applications to computer-assisted grading of cancer and image-based markers for prediction of cancer progression and survival. Rajpoot is a Senior Member of IEEE, and a member of the ACM, the British Association of Cancer Research (BACR), and the European Association of Cancer Research (EACR).
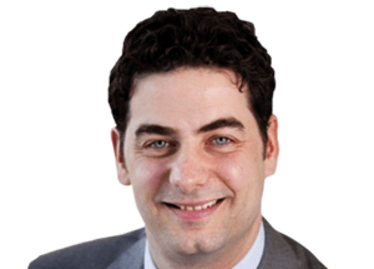
Dirk Vossen is Head of Strategy and New Business Development at Philips Digital Pathology Solutions. He is one of the founders of Philips Digital Pathology and has held multiple roles in Philips DPS. He has been responsible for product management, clinical studies and the first FDA clearance, and is currently responsible for partnerships and data collection for Computational Pathology. Dirk obtained a PhD in Physics (cum laude) on microscopy and optical micromanipulation in complex fluids.
What exactly is computational pathology?
Nasir Rajpoot: As a computer scientist first and foremost, but also someone with a great interest in the analysis of pathology images, computational pathology to me is simply the use of computer algorithms for understanding disease. The prefix “computational” does not change the meaning of pathology itself, which remains the study of disease and a way of understanding the status of a patient’s health. The “computational” aspect really relates to the emerging tools that enable us to achieve the goals of pathology in new, and perhaps better, ways. Clearly, my definition is broad. Delving a little deeper, computational pathology encompasses all kinds of algorithmic tools and analytics, and there is a lot of scope and excitement surrounding predictive modeling that allows some insight into the course of disease. That’s where the field is heading in the longer term.
Dirk Vossen: Nasir provides an excellent – and broad – definition with which I fully agree. But perhaps I can broaden it a little more by including some of the data elements and information from radiology, genetics and genomics in addition to histology and pathology in the narrower sense. So it’s very broad and it has a lot of potential. As a side remark, I feel like the field could actually benefit from clearer definitions that are accepted by the wider community – I hope we’ll start to see those in the coming years.
Jeroen van der Laak: I will add that I believe computational pathology is a means to pathologists’ becoming even more efficient and accurate in certain applications. Looking further into the future, as Nasir said, we will probably be able to extract information from tissue sections that really adds new layers of insight to routine pathology. Computers are able to extract visual information that is simply not accessible to the human eye.
What is the likely impact of computational pathology and healthcare – and in what timeframe?
JvdL: Well, healthcare is very broad, so I’ll stick to pathology and perhaps cancer diagnostics. Talking about timeframes is a little dangerous; when I started in pathology 25 years ago, there were people who used to say, “Within five to 10 years, image analysis will have taken over and the pathologist will be gone.” That said, I do think that, within five years, we will have computational pathology tools in the lab that support pathologists for very routine tasks, such as counting mitoses or finding metastases in lymph nodes. More elaborate tools that provide additional information to the process are more difficult to establish and assess, and will require changes to guidelines, so their implementation will take much longer. But you’ll certainly see the first algorithms in the next three to five years.
NR: I’d like to add that we are in the midst of a really exciting revolution in the domain of artificial intelligence (AI) and computer vision. There are many exciting developments, but also associated challenges. I agree with Jeroen that there is still plenty of low-hanging fruit out there. And if we can demonstrate the utility of computational pathology in those applications – and get them through the necessary approval processes – it will represent the field’s first real uptake. From then on, I believe the sky is the limit. And though we don’t want to over-hype the promise, the potential is very exciting.
The human brain is fantastic at recognizing people and understanding the nature of the world, but in histology, there are plenty of patterns hidden in plain sight that the naked eye cannot decipher. And I think that’s where computational pathology has a very important role to play. But as I said, relatively simple tasks – perhaps tasks that humans are good at, but find mundane – are perfect for reliable and robust systems. That’s why adoption in those areas will be faster.
DV: I agree with Nasir and Jeroen that the initial computational pathology applications will likely have clear benefits in terms of efficiency, enabling pathologists to get to the result or report faster. Here, we are talking about sorting, “finding the needle in the haystack,” pre-screening, and so on. Later, there may be completely new grading schemes and applications delivering information that today’s pathologists are not consciously able to see. I also agree that more advanced applications will take longer to develop; some are already in the research stage and look very promising, but it will still take time before they are ready for implementation. To be routinely used in the clinical environment, we need to move from the research to the proof-of-concept and finally to a product that can be used by everyone and anyone. So we need a controlled development phase, validation studies, and regulatory approval, all of which take a lot of time and resources. But I can say that it is happening right now.
Like Jeroen, I find it difficult to discuss specific timelines, but I would say that in the next 18 months you will start to see more applications based on newer technologies that will be integrated into the routine pathology workflow. However, I’d like to add one final important point to this part of the discussion: before you can start benefiting from computational pathology applications, you first need to go digital! Without digital pathology, there is no computational pathology. I consider it a two-step process.
Digital pathology already has its own clear business case. We know it works; we saw some great examples delivered at the Digital Pathology Congress of how a fully digital lab works. It’s no longer a question of whether or not it can be done, but a case of how and when. Taking the research that Nasir and Jeroen are doing – computational pathology – and bringing it into the clinical environment is the job of industry; we must prove the benefits.
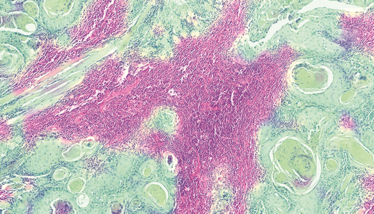
AI-based detection of tumor-infiltrating lymphocytes in oral squamous cell carcinoma.
Why will computational pathology assist, rather than replace, pathologists? In other words… do pathologists need to worry about their jobs?!
NR: I’m very clear in my mind about the answer to this question. Whatever the concept – digital pathology, computational pathology, AI pathology – it is never going to replace pathologists. I like to turn to the example of radiology, which was digitized 20 to 25 years ago; I don’t know a single radiologist who’s gone out of business as a result. In fact, there are many more radiologists today than there were before. If anything, I think computational pathology will excite the younger generation working through training right now. Uptake of digital pathology for training purposes is much higher than in clinical practice and routine diagnostics. Nobody should feel threatened by advances in digital and computational pathology. (Well, perhaps those people who refuse to even touch a computer should be a little worried…) Digital and computational pathology exist to assist. The integrative aspects of pathology, the cognitive abilities of pathologists, and the collective wisdom generated over many years as a community cannot be replaced by a machine. And speaking from a patient’s perspective, I personally wouldn’t want my sample being handled solely by a machine, even if it were possible – I would want any outcome sanity-checked by a human expert.
JvdL: I can add another interesting example: genetics. About 10 to 15 years ago, I remember people believed that, within a decade, we would only need to perform genetic analysis and that pathology wouldn’t be needed for many applications. Genetics would solve everything. In actual fact, the result of all this genetic analysis is that pathologists are confronted with much more data and more difficult questions in many cases. The integration of all this extra data and information requires a human expert, as Nasir says. Computational pathology can step in for some of the tedious tasks, but at the same time, it is likely to add even more information from a different angle for the pathologist to integrate into the whole picture. I believe the profession can only get more interesting.
DV: Computational pathology is an opportunity. Some will embrace it and make best use of the new tools available; others will take time to come round. But there is no threat unless you are completely unwilling to change the way you work. There is no doubt in my mind that the pathologist’s role will evolve – but the essential nature of that role will not change. The pathologist will be at the center of the diagnosis – and with growing importance.
I accept that digital pathology is unlikely to be a threat to pathologists – but what about the threat that molecular pathology poses to conventional morphological pathology?
DV: Jeroen already touched on this. There was an expectation by some that molecular pathology would make histopathology obsolete – but there is now a very different view: they are highly complementary. One is not going to replace the other. More interestingly, one can enhance the other. And computational pathology brings together the worlds of histology, digital pathology and molecular pathology.
JvdL: I fully agree. Sometimes, to interpret the results of molecular analysis, you need to know the composition of your tissue very accurately. And the tools that we develop based solely on morphology can really help you to better understand what the molecular signal is telling you.
NR: The keyword here is complementarity. No one should be afraid of another discipline taking over. Molecular pathology certainly has its place – but I think everyone now understands that it does not tell us the whole story; histology also plays a vital role. By combining the two, we create a more comprehensive picture, and I think that’s the way pathology is going. Pathologists will inevitably have to embrace other disciplines and be able to integrate signatures of disease from a number of modalities. There has been some really exciting work that shows how fusing different signatures gives you a more powerful marker than any one of those signatures alone.
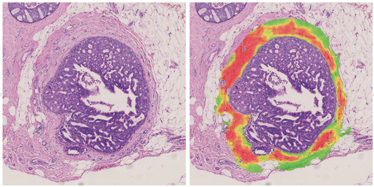
A computational pathology algorithm recognizes sub-visual morphological features, displaying alterations in the stroma that are associated with the ductal carcinoma in situ lesion.
As a pathologist, I’m happy to hear that the tools the experts are working on will assist and not replace us. But if you’re asking us to adopt computational pathology into routine clinical practice, I see a problem: we’re not data scientists. We don’t know how to interpret or validate the math behind your algorithms and we’re not trained in predictive modeling. How do we cross that divide and educate pathologists now and in the future?
DV: A super question. There’s a lot of development going on right now, much of it fundamental computational science research. It’s very complex, so you need a PhD in math or physics to even follow, let alone contribute, to it. You probably need to be a professor in the fields – like Jeroen and Nasir. So the fundamental research must be translated into the clinic, which means taking the basic knowledge and technology, showing that it works, and then building it into a product or tool. Such a product will not exist as an algorithm with certain performance curves and a lot of mathematics; instead, it will be developed to work in a practical way in the laboratory’s day-to-day workflow. The pathologist should intuitively know how to use such a tool, and when it is and isn’t appropriate to use. The inner workings of the algorithms – the “engines” of the product – don’t need to be, and will not be, exposed to pathologists. You would use the computational pathology system just as you would any other – but now with all that beautiful technology working behind the scenes to assist in your workflow. Pathologists will be able to rely on the quality of that algorithm not because they have delved into the processes themselves, but because it must be validated, reliable, robust and easy to use to exist at all.
JvdL: There is a whole area of research that we haven’t touched on yet. When you come to digital pathology conferences, you see people like us talking about our algorithms and the complexities of our research, but there are far fewer studies on how to successfully translate our findings into something that is useful to pathologists. We tend to stop when the algorithm gives the right results; we don’t study how to present this information to pathologists or how it will most optimally help pathologists with their workflows. I fully agree that, as a pathologist, you should not be concerned with what’s happening “under the hood” – just as with genomics, you’re unlikely to know exactly what is happening, and you may well not even run the PCR yourself. Even with a very good algorithm, if it’s presented in the wrong way to the pathology community, it could end up doing more harm than good to the workflow. Conversely, a less powerful algorithm could still help pathologists to a very high degree simply by making the workflow more efficient.
NR: I totally agree with my colleagues here. A nexus must exist between academia, the research community, and the commercial sector if we want to move some of these exciting algorithms into clinical practice. It can sometimes feel like the regulatory bodies are putting huge hurdles in our way – but it’s often for good reason, and we must remember that they exist to ensure that any approved algorithms are clinically validated. Consider a scanner for the imaging of your slides – you don’t need to worry about the electronics inside or the algorithms with which the circuits have been implemented; you just want to know that you’re getting what you were promised. In other words, you need to be assured that the algorithm can do its job in a reliable and robust manner.
Tools cost money. How much cost will computational pathology add to the workflows of a typical clinical laboratory – or, to put it another way, what value can it bring?
DV: This is a really good question that we get asked a lot! Who’s going to pay and how are we going to pay? In early research, there are always ways to find funding. When we enter the clinical space, such funding is no longer an option. Therefore, all of these tools will need to have a business case – either individually or as a suite. By business case, I mean justifying the benefits (value) that any tool brings to the lab versus the cost of not only purchasing those tools, but also implementing and running them. In the near future, you will start to see applications with positive business cases. One of the reasons we’re starting with tools that deliver efficiency gains is to make the business case as transparent as we can. I know that Jeroen has some good examples of how algorithms can help, and has delivered a number of presentations outlining the benefits.
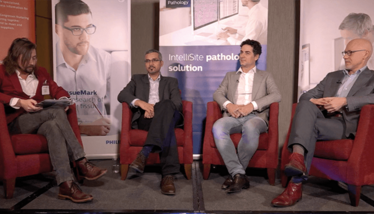
What will be the likely application areas to most benefit from computational pathology?
JvdL: The lowest of the low-hanging fruit is something that we’ve studied quite extensively: finding metastases in the lymph nodes of breast cancer patients – which I think is the example to which Dirk was referring. We have seen in pilot studies that we can bring the time required for a case down from 15 or 20 minutes to just five minutes, which represents a significant time saving for pathologists. Likewise, finding tumors in biopsies is another good example; you have to process many tissue sections in search of those that contain cancer. If the computer can identify the sections of greatest interest, it represents another time saving. Another well-studied example, which I’ve already mentioned, is counting mitoses for cancer grading. There have been many studies, again in breast cancer, in terms of counting mitoses or locating the spot to count for the best measure. Other targets include measuring the size of a tumor or measuring the distance to the surgical resection margins. These are the first simple applications that can be tackled with current technology. At a later stage, we’ll start looking at the grading of tumors using more sophisticated analyses.
NR: The list is rather long, as far as I know. I totally agree with Jeroen’s list, but I can also add things like immunohistochemical scoring, which can be problematic for certain cases, such as ER, PR and HER2. There are products out there already, and that’s because they add value by automating the relatively mundane tasks that don’t require a great deal of cognitive ability or experience to put a number on a slide. It’s been shown in our work and that of other labs that these tasks can be performed in a reliable manner – as well by a computer as by an average pathologist. But what’s really important going forward is the integration of these tools into the existing workflow, rather than disruption of that workflow. Anything that gets in the way of the pathologist and that does not result in cost savings is not going to be widely accepted; only those labs with deep pockets or high enthusiasm will move forward.
We’ve been hearing a lot about pathologists, our perspective on how these tools might be integrated, and what our needs are. I’m interested to know the needs of computer scientists. What do you need to achieve some of the objectives you’ve talked about – to get these low-hanging fruits into clinical practice?
JvdL: I think there are three levels to this. First, it’s important that pathologists identify where we can help them. We can build great tools, but we need pathologists to pin down exactly what is needed in their workflows. We may build a complex technical tool with a view to helping pathologists, only to find out that it’s actually a very simple task that does not benefit from automation. Conversely, trivial things at our end can be very helpful in a pathology workflow. Discussing potential use cases at length is essential.
Second, when we train systems, we need a lot of data and pathology expertise; making our algorithms as good as we want them to be involves a lot of work and requires a great deal of input. Third, once we have built the algorithms, we need pathologists to help validate them, which is essential from a regulatory point of view.
In short, we need help before, during, and after the development of computational pathology tools.
DV: I agree. We need collaboration between data scientists and industry, but we also need to find the right people – not only the visionary pathologists who have the time, space, and money to play with the technology, but also the people who have a real problem that can be solved with our tools. Digital pathology has been around for over 20 years as a technology and a promise, but it took a long time before the first labs made the decision to go digital – not 10 percent, not 20 percent, but 100 percent. These labs were run by very practical people with problems to solve – but also the expertise to work through the challenges associated with implementing new technology and new ways of working. And that’s what we need in computational pathology.
NR: I can see some of my students sitting in the audience and I can tell that they immediately know exactly what I’m going to say. What we really need from pathologists is input, and lots of it. Taking some of your experience for the kinds of tasks you can almost do in your sleep (metaphorically speaking) and translating it into something a machine can do is no small task. Other computer scientists will know that it has been – and is – hard to pin down colleagues in pathology. It can be difficult to find time to discuss our concepts at length, let alone provide the accurate annotations that we need to train some of our algorithms.
Computational pathology, by its very nature, is a multidisciplinary field, and we can’t do it alone. We need input from the related disciplines and it needs to be a joint effort. We’re not wizards who, with the help of AI, can make things
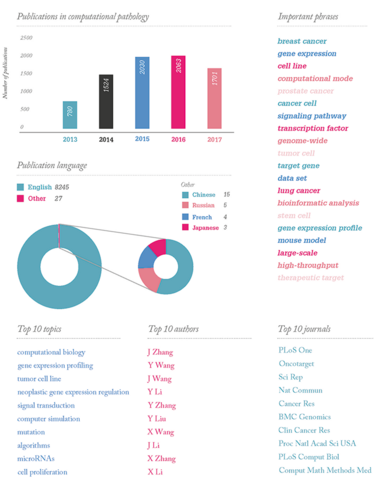
The questions and answers for this article were taken from a live online discussion session, supported by Philips: “The Benefits of Computational Pathology” that took place at Global Engage’s 4th Digital Pathology Congress: Europe in London, UK, in November 2017 (www.global-engage.com/event/digital-pathology).
To watch the full discussion, simply register for free (attendees qualify for 1 CPD point, issued by the Royal College of Pathology):
http://tp.txp.to/philips-webinar/TBCP
Jeroen van Laak is Associate Professor in Digital Pathology at the Radbound University Medical Center in Nijmegen, the Netherlands.
Nasir Rajpoot is Professor in Computer Science at the University of Warwick.
Dirk Vossen is Head of Strategy and New Business Development at Philips Digital Pathology Solutions.