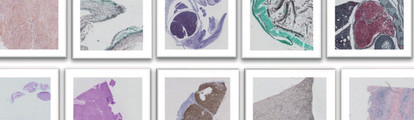
The Colorful Reality of AI
Why color standardization of whole-slide digital imaging is essential to reliable computer-aided diagnosis
At a Glance
- Color management is vital for many technologies – but none so much as histopathology
- Features of digital images, such as color, vary between scanners
- Because color is not standardized between devices and images, AI algorithms may not perform as accurately and reliably as they could with uniform input
- One approach to achieving uniformity involves the use of a special slide to standardize devices to real-world histopathology colors
Look around you and you’ll appreciate that “color management” is applied to a broad mix of everyday technology – from televisions to cameras; industrial label presses to cinema screens. Accurate, reliable color is deemed so important that these industries take extensive steps to mitigate any reduction in its performance or appearance. The integrity of color in medical images should be no different – in fact, given the implications of the technology, it’s where standards should be the most stringent of all!
Color critical
Different stains are applied to histology samples so that we can visualize important detail and specific tissue features using bright-field microscopy. Consequently, histopathologists use a wide range of colors to identify the presence of biomolecules, cells, and structures (see Figure 1). Tissue is precisely and selectively stained; the different colors are not deployed at random, but are selected to ensure effective diagnosis.
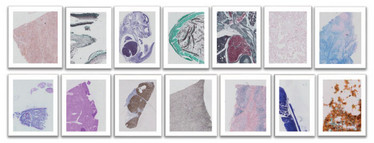
Figure 1: Histopathology has a wide range of colors that must be accurately digitized by WSI scanners.
The introduction of whole slide digital imaging (WSI) devices has great potential to improve medical diagnosis. However, each WSI scanner can be thought of as a unique arrangement of optical and digital components, which means that the digital image created varies from one device to the next. These differences are evident not just between manufacturers, or even across product portfolios, but even between individual scanners of the same model – a challenge that carries significant implications, including a lack of accurate, uniform color.
That’s why pathologists are calling for WSI scanner color to be measured and calibrated. As well as boosting users’ confidence, this will have positive implications for the development of high-accuracy digital pathology artificial intelligence (AI), with the ultimate goal of ensuring that data processed by computer-aided diagnosis and AI is standardized.
In fact, this issue is deemed important enough for both the UK’s Royal College of Pathologists and the US Food and Drug Administration (FDA) to include in their best practice recommendations for implementing digital pathology. In procuring WSI devices, the College states, “Pathologists may wish to include assessment of color accuracy in their testing” (1). The FDA goes one step further to detail how color differences between WSI devices should be addressed. Their recommendation reads, “The WSI system should be tested with a target slide. The target slide should contain a set of measurable and representative color patches, which should have similar spectral characteristics to stained tissue” (2).
The AI effect
Many factors have an impact on the accuracy of AI. Inconsistencies in data format and content reduce its ability to accurately categorize and quantify information, compromising decisions that must be accurate and appropriate. In the case of digital pathology AI, the potential impact of such inconsistencies could be, at best, delayed diagnosis and potential disease progression. At worst, it could result in misdiagnosis, ineffective treatment selection, and, ultimately, life-changing or even life-limiting consequences.
The different colors present in precisely and selectively stained human tissue are essential to accurate diagnosis. And that’s why it’s vital to not only train AI software to recognize spatial data, but also ensure the ongoing accuracy of input data and therefore decision-making reliability. This can be accomplished at least in part by working toward consistent, standardized color for digital images across WSI device types. It’s no easy task, especially when you consider that – despite knowing that color differs between WSI devices – data from multiple devices is used for the same AI/diagnosis. Until this issue is addressed, any analysis undertaken uses potentially compromised data. After all, we’re all aware of “garbage in, garbage out” – the idea that if bad data is put into AI systems, they will return equally bad results.
Some AI developers have adopted (or developed their own) workarounds to the computational handling of color inconsistencies. Those may work as stopgap measures – but why should laboratories divert brainpower, time, and resources to solving a fundamental issue that scanner vendors should address? Their time would be better spent focusing on the critical task of enhancing computer-assisted diagnosis.
Current approaches to color management
Interrogation of data at the machine-learning or deep-learning level requires a different approach to color interpretation. For instance, color segmentation can assess the approximate spread of color data in machine-learning applications, which allows users to address batch variation by normalizing individual stains to a custom color space. Within deep-learning AI approaches, histograms of individual color distributions are assessed at the pixel level for high-precision intra-image correlation. Such approaches can flatten and smooth color variation at the subcellular (non-spatial) level.
Although these methods offer fine control and measurement of color spread, they are limited by the extremes of data provided. Not only are the image input and the altered output likely to differ significantly, but neither is representative of the real, ground-truth tissue sample. Data is presented from intentionally and specifically stained samples to the AI to assist with diagnoses; bypassing or manipulating the color aspect could alter or entirely miss a piece of biological truth.
False coloring is most commonly used in image analysis to flatten variations between samples by artificially applying non-reality-standardized characteristics to cellular structures, which allows us to feed the AI uniform data. However, it is the most basic way of dealing with color and, in effect, ignores the existing color altogether. And so, all color-specific data (spectral truth, intensity, and density) and the biology it represents is compromised. Instead, the focus is exclusively on the spatial data of tissue structures – meaning that we have less than 100 percent of the available data and, worse yet, less than 100 percent of the data we need for a complete diagnosis.
Emerging techniques
Newer and more specific techniques have emerged, aimed at increasing the accuracy of computer-assisted diagnosis. The goal is to allow universal AI on images from differing clinical and scanner sources. Of particular note is the translation of color from pre-existing applications by Cycle Generative Adversarial Networks (CycleGANs).
In Figure 2a, a CycleGANs image algorithm has been used to maintain the spatial information of a zebra and orange (representing cells and tissue, respectively) while converting color to simulate an image of a horse and apple (synonymous to digitally converting specific stain color) (3). In Figure 2b, the same technique has been applied to a mountain landscape, changing it from a summer to winter scene. In this context, the algorithm could be used for marketing purposes such as tourism to make the same location influence different traveler personalities. “Counterfeiting” images in such a way could be considered misleading – but, in advertising, communication, or media arenas, that carries only minor implications. In medical diagnostics, on the other hand, actively manipulating an image containing medical data prior to diagnosis has significant implications because you’re altering life-critical data.
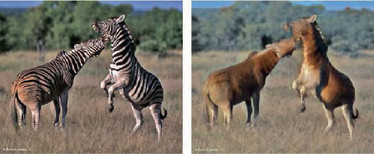
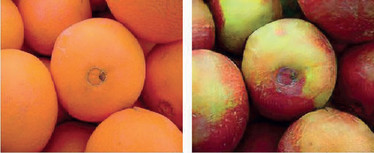
Figure 2. a) Zebras and oranges (“tissue”) converted to horses and apples (“staining”) by CycleGANS.
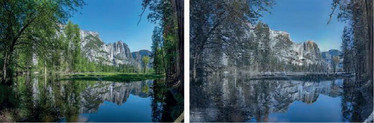
Figure 2. b) CycleGANS can influence perception of an image, transforming a serene lakeside retreat into a challenging mountain to climb.
From a practical point of view, emerging evidence indicates that applying CycleGANS to tissue images from multiple scanner types improves the accuracy of pre-existing AI tools. This technique could normalize colors received from different scanners to a standard color space, rather than making them appear as something different entirely. However, different algorithms work to different accuracies (4), meaning that this methodology is still susceptible to high degrees of variation and that its output is still artificially constructed. Ethical issues aside, the fact that simply changing the color of scanner X images to match those of scanner Y results in increased AI accuracy indicates that color is a significant influencer on digital diagnosis.
This recognition of color’s importance for refining AI accuracy is met by the recent development of color-standardizing algorithms that can be used as a pre-diagnostic method (5). These create a deep-learning color-handling tool for analysis, using extensive, pixel-level color and spatial analysis to separate the specific stains co-located in a single pixel. They are then combined with established chromatic data for each stain component, allowing the application of hue/saturation/density relative to the entire WSI from the pixel level. The result? A universal standardization algorithm that has the potential to be applied in combination with developed (or developing) AI tools to generically increase computer-assisted diagnosis accuracy through digital color management.
This major step forward recognizes and mitigates the effect of color on the pixel, spatial, and cross-sample level of big data analysis – but it’s not the end of the road. The core definition of color is still from inferred data only, and doesn’t encapsulate the absolute truth held within each tissue sample. Absolute color truth in WSI images can only ever be addressed by a direct, real-world, scanner-specific measurement.
A firm grounding in reality
A key message emerges from these different AI approaches: color uniformity and reliability are important for maximizing AI accuracy. Although there is no denying that spatial data on cellular distributions in tissue is the main driver for all diagnosis, it may not completely dominate the computer-assisted diagnosis landscape – as evidenced by CycleGANs, where transformation to a standard color by scanner-to-scanner mimicry “flattens” color variation.
Such a realization may not seem significant at first; after all, if color is the only thing that changes, we maintain the inevitable image quality variation – focus, stitching, and resolution – that arises from using two different imaging systems. But, even though the differences in non-color data quality should negatively affect analysis if spatial data were the only driver, it appears that CycleGANS enhance, rather than diminish, AI accuracy.
As effective as post-imaging data-handling techniques may be, none fully embrace the FDA’s recommendations, nor do they enable AI solutions to use “ground-truth data” that captures all scanner-to-scanner, image-to-image reality. When the original color is consistent between scanners, we’re likely to see even further improvement.
An alternative approach is to use International Color Consortium (ICC)-standardized color profiles to calibrate WSI devices. This method involves using a slide with a number of histologically stained patches that precisely mimic stained tissue to measure the spectral absorption of commonly used pathology stains. Measurements are then combined with each scanner’s color interpretation of the same slide and the interpreted error (the scanner’s individual deviation from a standard truth) calculated. ICC profile metadata is then generated and used to correct the pre- or post-imaging output so that the digital image contains ground-truth color.
The color is not only closer to truth, but also unified across all scanners. For AI software, the key advantage is the application of such calibration technology on a regular basis to ensure that decisions are made based on uniform input, and that all images, irrelevant of source, remain digitally unaltered and are quality-controlled to be ground truth color as standard.
To demonstrate the impact of color management using this ground-truth calibration slide method (6), color error can be reduced by a factor of more than 700 (see Figure 3), which raises two big questions. First, this is medical technology – is it acceptable for a medical device to have more than 700-fold error in any aspect of its function? And second, the downstream use of AI is human-free – do we trust decisions made on data that either has 700-fold error in a major component or is artificially manipulated by algorithms to satisfy a successful outcome?
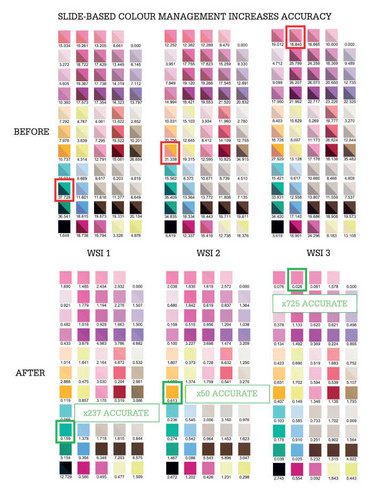
Figure 3. Errors in color before and after slide-based color management of three different WSI scanners from different vendors. In some regions of the gamut, color error was reduced by 700-fold or more.
Within the lifetime of anyone reading this article, medical imaging and AI have made huge advancements toward automation. To achieve this degree of computer-assisted diagnosis accuracy and reliability, we have liberated more layers of data buried within each WSI. However, AI consequently requires increased data quality and validation stringency. The discrete color of a tissue sample represents the molecular-level interactions at which refined pathology with cutting-edge precision can be performed. AI’s ability to cope reliably with color variation between WSIs and to create a uniform interpretation of these differences has shown clear improvements in preclinical AI, increasing its application to large and varied datasets.
Color management is a fine-edged tool that makes AI commercially competitive and reliable. But AI still has its limitations; any computational color-handling is no more than data manipulation with varied degrees of success. The ground-truth colors in pathology are real data from real patients who require diagnostics based on reality. Only by using color management techniques with the ability to create standardized, ground-truth colored images irrespective of WSI scanner source will AI truly be able to offer complete color certainty to assist with life-changing medical decisions.
There’s no denying that the AI future is coming… but when it comes to the ultra-precision offered by color management, AI must be firmly grounded in reality.
- S Cross et al., “Best practice recommendations for implementing digital pathology” (2018). Available at: bit.ly/2MvpO7c. Accessed July 31, 2019.
- N Anderson, A Baldano, “Technical performance assessment of digital pathology whole slide imaging devices” (2016). Available at: bit.ly/310Uscq. Accessed July 31, 2019.
- J-Y Zhu et al., “Unpaired image-to-image translation using cycle-consistent adversarial networks” (2018). Available at: bit.ly/2N30uXy. Accessed July 31, 2019.
- MT Shaban et al., “Staingan: stain style transfer for digital histological images”. Presented at the IEEE 16th International Symposium on Biomedical Imaging; April 8–11, 2019; Venice, Italy.
- B Ehteshami Bejnordi et al., “Stain specific standardization of whole-slide histopathological images”, IEEE Trans Med Imaging, 35, 404 (2016).
- EL Clarke et al., “Development of a novel tissue‐mimicking color calibration slide for digital microscopy”, Color Res Appl, 43, 184 (2017).
Richard Salmon is Product Manager for Life Sciences at FFEI, Hemel Hempstead, UK.