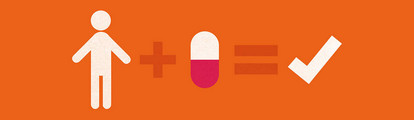
Predicting the Impact of Personalization
Kinetic models allow researchers to integrate multiple physiological responses into a single test that predicts patients’ likelihood of drug side effects
When you think of personalized medicine, what comes to mind first? If you’re like many people, you envision a discipline designed to help doctors select the most effective course of treatment for a given patient. Understanding a patient’s molecular profile allows therapy to be tailored to a patient’s specific risk and response factors. But drug efficacy isn’t the only purpose of personalized medicine – it’s equally important for ensuring a treatment’s suitability, including the potential for side effects. That vital function is what inspired us to develop a new model for predicting the side effects of drugs, based on genetic and metabolomics measurements taken from patient blood samples.
At a Glance
- Personalized medicine currently relies heavily on single-factor tests such as enzyme or metabolite measurements
- While vital to progress in precision medicine, such tests don’t account for the complex behavior of multifactorial systems like cells or whole humans
- Whole-cell kinetic models integrate genomics and metabolomics to describe cell properties and predict patient outcomes
- These models are not yet clinic- ready, but may one day be powerful tools in personalizing patients’ treatment plans
The building blocks of kinetic models
The first step in our process was to select a cell type to serve as a test subject for our model. We chose the humble red blood cell. This was in part because it’s the simplest available human cell, in part because it’s so easily accessible from blood samples, and in part because researchers and healthcare providers have a tendency to underestimate the role that these cells play in systemic health and disease processes – including drug metabolism. Both our group and others have a long history of building models of the human erythrocyte to simulate and analyze enzyme deficiencies (1)(2). In some of our earlier work, we noticed that these models were able to identify severity of enzyme deficiencies, such as glucose-6-phosphate dehydrogenase (the first enzymatic step in the pentose phosphate pathway) and predict the severity of a patient’s susceptibility to hemolytic crisis (by examining their single-nucleotide polymorphisms) (3).
Given the findings from these previous studies, we hypothesized that individual specific models could be constructed using metabolomics profiling from volunteer participants’ plasma and red blood cells (4). These were whole-cell kinetic models of erythrocyte metabolism, and we constructed them for 24 healthy individuals – based both on fasting-state plasma and erythrocyte metabolomics and on whole-genome genotyping. By gathering and incorporating both genetic and metabolic information, we were able to use the models to link genotype to protein function. Ultimately, we demonstrated that differences in patients’ personalized kinetic rate constants were reflective of differences in physiology – allowing us to spot patients at risk for drug side effects. In our paper, for instance, we used our models to identify individuals at risk of ribavirin-induced anemia, then also identified a particular genetic variation, inosine triphosphatase deficiency, that may protect against it. Constructing similar kinetic models for other patients may give us the ability to characterize individual metabolic variation – helping us choose the most effective and least harmful drug for each patient.
Weighing drug risks and rewards
There’s no single biggest issue with individual variation in drug response. Rather, it’s largely dependent on the disease being treated and the available medications. For example, at the moment there is a big push in oncology to move toward more personalized treatments, so of course inter-individual responses to medications is of prime interest. That’s not to say that there isn’t also a concern regarding adverse effects – but in many cases, those are anticipated and can be treated. The goal in furthering our understanding of and ability to predict inter-individual variation in drug response is to help define the risk/benefit ratios of prescribed drugs with greater specificity.
Of course, it’s impossible to look at risk/benefit ratios without considering both sides of the equation – and that’s where side effect investigation comes in. We need to identify patients with different susceptibilities, because the potential severity of a treatment’s side effects can have a significant effect on doctors’ ultimate decisions.
The computerized cell
To explore the pros and cons of treatments in individual patients, we created an “in silico cell” that reflects erythrocyte metabolism. We then applied data to this in silico model and observed its behaviors as a prediction of how a red blood cell would react to treatment in vivo. Our approach uses direct metabolomics data to generate individual specific models of metabolism for the cell. These models simulate the way patients’ erythrocytes will react to various conditions – in our case, tolerance to different doses of drugs like ribavirin.
Building the model is a multi-step process (see Figure 1). We started with our pre-existing knowledge of the enzymatic proteins and the biochemical reactions that occur in red blood cells – the biochemical network. Next, we collected metabolomics data by carrying out a series of small molecule measurements from the plasma and red cells of healthy individuals. We used these data to construct a differential equation model based on mass action kinetics (Mass Action Stoichiometric Simulation, or MASS models), from which kinetic parameters from each individual can be calculated. Conceptually this approach differs from some of the standard approaches for building models in that, rather than taking a series of measurements and fitting a model to the data, we move in the reverse direction and from a set of measurements we calculate parameters that satisfy constraints on the system (mass conservation, reaction directionality, etc.). There is an algorithmic procedure for the steps in the model construction pipeline, so although we still have a few steps that require expert curation, much of the process may eventually be automated.
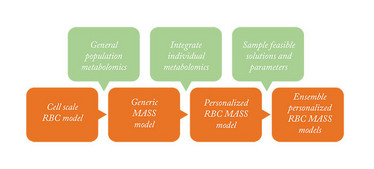
Figure 1. Generation of a MASS personalized kinetic model for erythrocyte behavior. The model allows investigators to predict red blood cell reactions to various conditions, such as drug treatments. MASS: mass action stoichiometric simulation; RBC: red blood cell.
From byte to bedside
Initially, we didn’t expect to identify individuals with potential susceptibility to treatment side effects. Our goal was just to determine if we could differentiate between patient populations based on metabolic differences, and link these differences to the individuals’ underlying genetics. Models aren’t the only way to do this – supervised and unsupervised learning methods have been successful at differentiating samples from different individuals. But the advantage of models is that they have biological coherence, which means that we can interpret the observed behavior and predictions in the context of the metabolic network. Models also allow us to explore specific hypotheses for the observed results – that is, after we see the model’s predicted outcomes for a particular manipulation, we can use it to investigate the potential causes of those outcomes. The results of our initial study suggest that it’s possible to apply this methodology to other cells in order to achieve personalized diagnoses and treatment plans.
That’s not to say that it’s ready for the clinic just yet. For clinical use, there would likely need to be simplified versions of the models targeted at capturing only the features necessary for a specific application (like spotting potential adverse reactions to a particular drug). Such models would also enable simultaneous interpretation of multiple measurements – so, for instance, doctors who order metabolite panels won’t have to struggle to consider the implications of 10 or more variables at once; instead, they can simply use the model to integrate the data and provide a single “functional” interpretation. At the moment, the model we’ve developed is a general one that can accommodate multiple variables, but that adaptability makes it too complex for easy clinical use. With time, though, we can design models that address a single test’s parameters – making them useful tools for refining personalized patient care.
Kinetic models like this are only just beginning to find a place in laboratory medicine. The possibilities for exploration are still wide open, but for us, the next step will be to further test our models’ predictions of susceptibility to drug side effects. We’d also like to expand the model to include other cell types, such as platelets and hepatocytes.
With the ability to integrate multiple “-omics” into a single source of information, kinetic models may soon be the tool of choice for studying variations in physiology and drug responses – and we hope that, one day, they will become a standard part of patients’ personalized treatment plans.
- TA Rapoport et al., “The regulatory principles of glycolysis in erythrocytes in vivo and in vitro. A minimal comprehensive model describing steady states, quasi-steady states and time-dependent processes”, J Biochem, 15, 449–469 (1976). PMID: 132930.
- N Jamshidi, Palsson BO, “Systems biology of the human red blood cell”, Blood Cel Mol Dis, 36, 239–247 (2006). PMID: 16533612.
- N Jamshidi et al., “In silico model-driven assessment of the effects of single nucleotide polymorphisms (SNPs) on human red blood cell metabolism”, Genome Res, 12, 1687–1692 (2002). PMID: 12421755.
- A Bordbar et al., “Personalized whole-cell kinetic models of metabolism for discovery in genomics and pharmacodynamics”, Cell Syst, 1, 283–292 (2015).
Neema Jamshidi is a visiting scholar with the Systems Biology Research Group at the University of California, San Diego, USA.