In Other News: Digital Pathology
A quick-fire roundup of the latest in digital pathology developments
George Francis Lee | | 2 min read | Future
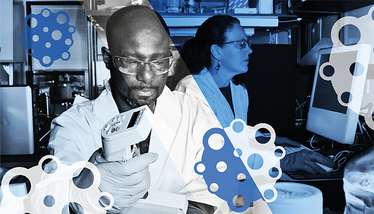
iSpy
To create a less resource-intensive alternative to transformers, researchers have created DPSeq – a digital pathology classifier capable of predicting cancer biomarkers (PMID: 37775043). DPSeq was constructed using H&E colorectal cancer images from The Cancer Genome Atlas and Molecular and Cellular Oncology datasets and demonstrated high accuracy in detecting key colorectal cancer biomarkers, including BRAF and TP53 mutations. It was also able to detect hypermutation, as well as microsatellite instability and CpG island methylator phenotype status.
DP and the ink machine
Automated scanning of the cell block technique can prove problematic, with incomplete scans leading to difficulties and inaccurate diagnosis. Researchers have tested the viability of overcoming this workflow hurdle by inking cell blocks prior to scanning (PMID: 37724610). Cell blocks were used in two tests: one in which blocks were inked one half green and one half black; and a second test where one half was black and the other unstained. The team found that, although inking required additional time and larger amounts of data, the method increased automatic detection by the scanner after immunostaining.
TLC for ILC
Some forms of breast cancer, such as invasive lobular carcinoma (ILC) are difficult to detect with mammogram methods. Now, researchers have tested whether digital mammography and digital breast tomosynthesis can improve detection and patient outcomes (PMID: 37697031). The study pulled data from over 830,000 cases in the Breast Cancer Surveillance Consortium and followed examinations for up to a year. The team discovered that digital breast tomosynthesis showed greater cancer detection in ILC, alongside invasive ductal carcinoma and invasive mixed carcinoma.
Patient prediction
There is a known link between pathological complete response to neoadjuvant chemotherapy and patient outcomes in cases of breast cancer. Unfortunately, the rates of pathological response can sometimes be below 30 percent with certain forms of the cancer. With this in mind, researchers used deep learning to predict outcomes of breast cancer chemotherapy using digital histology slides of pre-treatment biopsies (PMID: 37403567). The chemotherapy response was calculated for 207 patients through a hierarchical deep learning framework made up of convolutional blocks and self-attention modules. “The results of this study pave the way toward a response-guided therapy paradigm for individual breast cancer patients and motivate future studies on larger multi-institutional datasets for further investigation of the proposed methodologies,” concluded the authors.
Learning from experience
Case study of total transition to digital slides in six hospitals spotlights leaps in scanner capabilities in a short timeframe (PMID: 38142526).
Survey says
A survey of UK Liver Pathology Group members shows overall positive attitude towards digital pathology and AI in clinical practice (PMID: 36599660).
Deep infiltration
Neural network analysis of primary melanoma whole-slide images shows improved uniformity and reduced pathologist monitoring, with promise for routine use (PMID: 37734590).
Practice makes perfect
Self-supervised learning tasks relevant to lung adenocarcinoma subtype classification help neural network model better detect salient features in slide images (PMID: 37741228).
Credit: Images for collage sourced from National Cancer Institute via Unsplash.com
Interested in how disease interacts with our world. Writing stories covering subjects like politics, society, and climate change.