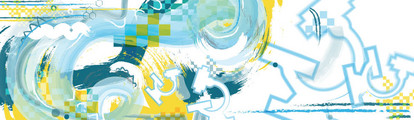
(AI) Trial and (Diagnostic) Error
How can new healthcare technology help address the number one patient safety issue?
At a Glance
- Diagnostic errors were recently named the number one issue in the 2019 ECRI Patient Safety Concerns report
- Artificial intelligence (AI) can help to detect and mitigate these errors
- Using AI for image analysis frees up time for pathologists to carry out the tasks that require the most skill
- Broader adoption will help produce real-world evidence supporting the role of AI in improving patient safety
The Emergency Care Research Institute (ECRI) recently published their 2019 patient safety report outlining the top 10 concerns affecting patients across the continuum of care. Using information from their patient safety organization database, root cause analyses, and votes from a panel of experts, the ECRI creates the list to help healthcare organizations identify and respond to new patient safety threats.
For the second year running, the 2019 report names diagnostic error as the number one patient safety issue, stating, “When diagnoses and test results are not properly communicated or followed up, the potential exists to cause serious patient harm or death.” More specifically, the briefing scrutinizes the management of test results using electronic health records. “Providers have begun relying on the electronic health record (EHR) to help with clinical decision support, to track test results, and to flag issues. However, the EHR is only part of the solution,” the report says.
Diagnosing diagnostic errors
To understand how we can combat and prevent diagnostic error, it’s important to appreciate how and why they occur in the first place. We don’t understand everything about every disease process – and what we do understand is based on our knowledge of the average person. But, as diagnosticians are all too aware, disease is highly personalized and influenced by a plethora of factors – so what holds true for one patient may be inaccurate for another.
The term “diagnostic error” can describe a range of mistakes, from a wrong assumption due to incorrect self-reporting from a patient to the use of an unsuitable diagnostic test. The multidisciplinary aspect of modern medicine – although beneficial in many ways – can sometimes cause complications because of the huge team of doctors who need to work together to make a diagnosis. Overdiagnosis is also a major issue and often results from the unnecessary use of diagnostic tests, which increases the number of patients who receive a false-positive result.
As a result, achieving the correct diagnosis 100 percent of the time is almost impossible. Diagnostic errors are an inevitable consequence of the heterogeneity of human disease and, rather than attempting to prevent them altogether, the most effective strategy is to detect, monitor, and mitigate these types of errors to minimize their impact on patients. And that’s where a range of emerging healthcare technologies can play a crucial role.
The AI solution
One of the ways that technology can alleviate diagnostic error is with image analysis, in particular with the application of artificial intelligence (AI) to the analysis of certain assays. Cases in which a diagnosis is dependent on a quantitative score or count, such as estrogen receptor positivity or Ki67 count, require little skill but demand a disproportionate amount of a pathologist’s time. If we can remove these mundane tasks from pathologists’ workloads by using AI to make quantitative determinations from slide images, we will free up more time for crucial, highly skilled tasks that aren’t easily replaceable by technology.
Another benefit comes in the form of time to diagnosis. The image analysis can run on a computer in the background and the pathologist can pull up the case when complete and instantly read the results. Increasing throughput in this way ultimately leads to more timely diagnoses for patients. AI can also help in terms of subspecialty staffing – when the technology can begin to help make diagnostic calls and triage cases for review by the pathologist, workloads will become more manageable and precious resources can be more efficiently directed.
But how do the benefits promised by AI translate to more accurate detection and better mitigation of diagnostic error? One of the strengths of AI is its ability to be implemented to detect and flag discrepancies. It’s also well documented by now that the combination of doctor and AI is more accurate than either on its own. Therefore, a combination of both human and AI expert systems can reduce misclassifications and prevent the potentially harmful ramifications of misdiagnosis for the patient. In addition, AI systems are not sensitive to burnout, reducing the risk of human error that comes naturally from exhaustion. This, coupled with the fact that AI systems can complete tasks to a consistent standard regardless of location or time of day, makes AI an attractive prospect for addressing diagnostic errors.
A question of trust
It has already been shown that AI can perform as well as, or even better than, the pathologist – but this depends on the type of task undertaken. For example, if it’s a binary call (such as the presence or absence of disease), pathologists can make that decision quickly and with high concordance between different individuals. If it’s a more quantitative grading of disease severity or the crucial task of outcome prediction, the performance of AI has the opportunity to exceed that of the average pathologist in terms of speed and accuracy. In cases that demand a large amount of quantitation with high levels of consistency and accuracy, pathologists already begin to lose out to AI, simply because the human eye and brain have not evolved to score consistently and precisely over long periods. The more this type of task is needed, the more support AI can offer.
Given this great potential, it’s fair to wonder why diagnosticians haven’t yet fully embraced AI. The answer to that question lies in a uniquely human attribute: trust. Although there is a wealth of evidence that AI can perform specific image analysis tasks accurately, questions about safety and generalization remain. If we train an AI model on a patient population in Boston, will it work effectively on patients in Amsterdam? Pathologists have excellent localized knowledge and better risk awareness than AI systems; they can spot immediately when something appears odd or different to the norm. AI systems struggle to recognize when something is amiss – especially when we force them to make a call solely on the presented image.
There are also issues with patient demography, which occur if the composition of patient populations changes over time. Because it’s impossible to validate AI systems against the entire human population, they can’t address all demographic changes without human intervention. AI is still an emerging tool and, although we are very good at understanding where humans fail – and have systems in place to detect and reduce the impact of those failures – we are yet to fully appreciate the ways that AI can fall short.
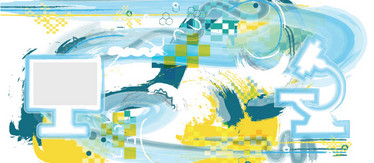
Indirect impacts
There’s only one way to further our understanding of the advantages and limitations of AI – and that’s through widespread adoption of the technology in clinical research and, eventually, clinical diagnostic settings. Only then will we be able to obtain real-world, parallel evidence about AI’s capabilities compared with those of humans. It’s vital that this data comes from pathologists and not from stakeholders using AI for academic projects, which are not always reproducible, scalable, or transferable to patients. Only by using AI in a controlled and safe environment can pathologists begin to gather experience and expertise. As with all new technologies, early adopters will pave the way for everybody else.
However, it’s important to assess the impact of AI in the correct way, especially as many of its benefits will be seen indirectly. AI systems might not improve health outcomes rapidly in the short term; although they will speed up certain tasks, that won't, in itself, necessarily improve diagnostic accuracy. Instead, it will free up time for the pathologist to carry out more complex, non-AI-based tasks that will impact health outcomes. The fact that such benefits are indirect consequences of AI assistance makes it difficult to quantify AI’s involvement in the improvements to health outcomes.
To effectively measure the impact of AI, we will need to take a wide range of criteria into account: direct measurement of AI’s performance, white paper economic analyses, and in-depth reviews of overall diagnosis rate. For example, do hospitals that adopt AI see – with the same staffing levels – an overall drop in problematic diagnoses? Do they see higher turnaround and better health outcomes than before the introduction of AI? It’s a complex metric to assess, but there are ways it can be done.
AI’s bright future
There’s still a range of feelings among pathologists and laboratory medicine professionals toward AI in diagnostic medicine. Some people are staunch in their view that it’s the doctor’s responsibility to make the diagnosis (and not the computer’s). I believe that is true, and that it will remain the case for a long time to come, regardless of new technology. Human disease is so complex that the fear of an AI system’s taking over and replacing humans is hugely unrealistic. Physician burnout is much more of a concern than the takeover of AI and, until we are at the point where diagnosticians don’t have enough work to do, it’s more important to support pathologists with incredibly busy workloads in any way we can.
The big question surrounding AI is not whether it can perform as well as humans; rather, it’s whether AI systems can carry out tasks safely at large scales. Many non-health-based fields are ahead of pathology in terms of adopting AI but, in those cases, mistakes aren’t as costly. In healthcare, even the smallest mistake can have serious consequences for patients – and that’s why we have regulatory oversight on everything we do. The next hurdle for AI will be to prove that it can deliver safely across a large, variable patient population.
AI holds great promise for the future of medical diagnostics, especially when it comes to spotting and reducing diagnostic errors. Ultimately, both patients and pathologists will benefit from the widespread adoption of AI – and, thanks to faster time to diagnosis and safe, robust human-AI partnerships, diagnostic errors will hopefully become less prevalent.
Senior Scientist at Aiforia Inc, Boston, USA.