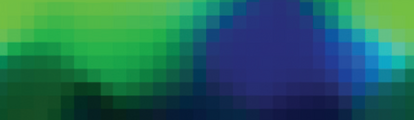
With a Little Help from AI
Improving artificial intelligence for leukemia and lymphoma diagnostics

Credit: Max Zhao.
When it comes to artificial intelligence (AI) diagnostics, there are discrepancies between labs – with smaller standalone labs often yielding too little data to train highly accurate AI models. For leukemia and lymphoma diagnostics, multiparameter flow cytometry (MFC) is a vital tool in the pathologists’ toolbox; however, protocols are subject to change depending on individual labs’ diagnostic workflows and available cytometers. Could the two methods help each other out?
A group of researchers at the University of Bonn have combined transfer learning (a machine learning technique) with MFC to improve the robustness of AI in leukemia and lymphoma diagnostics (1). “The gold standard is diagnosis by hematologists, which can also take into account results of additional tests,” said Peter Krawitz of the Institute for Genomic Statistics and Bioinformatics at the University Hospital Bonn (2). “The point of using AI is not to replace physicians, but to make the best use of the information contained in the data.”
The workflow takes AI models that are trained on a specific MFC panel and extends them to multiple MFC panels and data sizes. The team found that this extension improved AI performance and achieved high accuracy for multi-label classification of hematological malignancies across datasets. The workflow also enabled models to quickly adapt to changes in the data – making it accessible for a variety of routine diagnostic settings. Though the model’s diagnosis is only a suggestion and requires physician verification, lead author Nanditha Mallesh noted that “AI takes full advantage of the data and increases the speed and objectivity of diagnoses (2).”
The researchers have made the data and software freely accessible – and their collaborators at res mechanica GmbH have created a web service to make AI more accessible to users who lack bioinformatics expertise. Hannes Lüling, founder and CEO of res mechanica GmbH, stated, “We want to enable the exchange of anonymized flow cytometry data between laboratories and in this way create the conditions for even higher quality in diagnostics.”
- N Mallesh et al., Patterns, 2, 100351 (2021).
- University of Bonn (2021). Available at: https://bit.ly/3BLQOWw.
During my undergraduate degree in psychology and Master’s in neuroimaging for clinical and cognitive neuroscience, I realized the tasks my classmates found tedious – writing essays, editing, proofreading – were the ones that gave me the greatest satisfaction. I quickly gathered that rambling on about science in the bar wasn’t exactly riveting for my non-scientist friends, so my thoughts turned to a career in science writing. At Texere, I get to craft science into stories, interact with international experts, and engage with readers who love science just as much as I do.