
Unraveling Complexity
A new deep learning model may yield novel associations between genes and diseases
If there’s one thing we are learning from our ever-increasing fount of genetic knowledge, it’s that the relationships between genes and diseases are often complex. The more mutations we find and the more genes we associate with particular diseases, the more tangled the web appears. But with thousands of biomarkers available to us and new ones discovered all the time, how do we determine which are truly causative, which associated, and which merely coincidence?
A team of researchers from King Abdullah University of Science and Technology is harnessing the power of artificial intelligence to do just that, with a new deep learning model that the researchers say outperforms existing methods. They call their approach GCN-MF: graph convolutional networks and matrix factorization (1).
A graph convolutional network is a deep learning model that, in this case, analyzes how genes network and the similarities among genetic diseases. The resulting data is then organized into matrices that yield further information on gene–disease associations. Unlike existing methods, which are based on linear models, GCN-MF can examine nonlinear associations to yield brand-new information about the genes involved in complex diseases.
“By making use of more information, we achieved better accuracy than the state-of-the-art methods currently in use,” first author Peng Han said in a recent press release (2). He cautions, though, that the model isn’t yet ready for prime time. First, he and his colleagues need to incorporate new types of data and challenge it with new types of problems – and then, hopefully, GCN-MF can make its debut in the clinic.
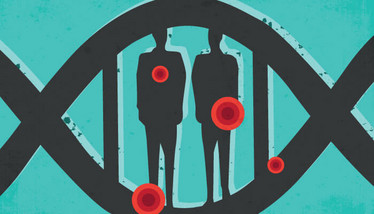
- P Han et al., “GCN-MF: disease-gene association identification by graph convolutional networks and matrix factorization”. Presented at the 25th ACM SIGKDD Conference on Knowledge Discovery and Data Mining; August 6, 2019; Anchorage, USA.
- KAUST Discovery, “AI learns complex gene-disease patterns” (2019). Available at: bit.ly/31UEHEK. Accessed October 7, 2019.
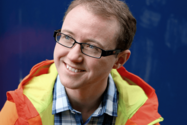
While obtaining degrees in biology from the University of Alberta and biochemistry from Penn State College of Medicine, I worked as a freelance science and medical writer. I was able to hone my skills in research, presentation and scientific writing by assembling grants and journal articles, speaking at international conferences, and consulting on topics ranging from medical education to comic book science. As much as I’ve enjoyed designing new bacteria and plausible superheroes, though, I’m more pleased than ever to be at Texere, using my writing and editing skills to create great content for a professional audience.