The Search for Next Gen Lung Cancer Diagnostics
Research discoveries continue to improve the outlook, but how promising are they?
Early-stage lung cancer is a diagnostic challenge; symptoms often don’t develop until the disease is advanced, and widespread screening is not currently an option. Since faster detection is key to improved survival rates, finding better ways to catch and categorize lung cancer would be a boon to oncology. Unsurprisingly, the search for diagnostics is a hotbed of research and here we report on two teams who are shedding new light.
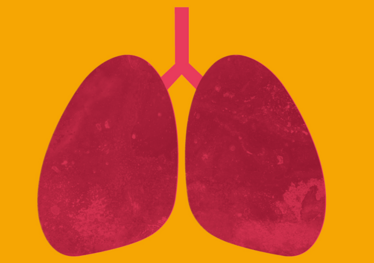
The first, a team headed up by Gerard Silvestri of the Medical University of South Carolina, USA, has been on the search for a diagnostic method that can be used alongside bronchoscopy to improve diagnostic rate. Their potential solution: a genomic classifier. “We needed better tools to predict whether lung lesions detected on X-ray or CT scan were cancer. Our minimally invasive procedures were not always giving us answers, requiring us to perform invasive surgery, and for some patients this turned out to be unnecessary,” explains Silvestri.
A genomic classifier based on the expression of 23 genes and patient age was tested on respiratory epithelial cells collected from current and former smokers undergoing bronchoscopy for suspected lung cancer. The rationale for using the classifier was firmly justified when Silvestri and his colleagues found it to improve the rate of detection from 75 to an impressive 97 percent (1). Speaking of its potential applications in the clinic, “In patients with moderate risk of cancer, a non-diagnostic bronchoscopy and a negative genomic classifier would provide a safe means of serial imaging, rather than moving to surgery,” says Silvestri.
For cases where biopsy does becomes necessary though, a second team from Ruhr University Bochum, Germany, has developed a fast, non-destructive and automated system for classifying cancerous tissue. The technique, named spectral histopathology (SHP), uses vibration spectra on lung biopsy sections to identify cancerous changes.
Because it relies on computational analysis of the spectra, no dyes are required, and tissue can be reused for H&E staining or molecular testing. A reference database, compiled with the input of pathologists, is used to automatically allocate the spectra to the matching tissue type, helping to classify tumors and predict disease aggressiveness. So far, SHP can identify all tumor classes, and distinguish prognostic subtypes of adenocarcinomas.
How effective is it? A study of 101 patient samples classified using SHP showed 97 percent accuracy when classifying tumor type, and 95 percent accuracy in subclassifying adenocarcinoma (2). The researchers now hope to further validate and develop the project with a larger, independent study.
What does this mean for pathologists? SHP has several potential, relevant applications – spectra analysis could be used alongside traditional staining to give pathologists a “second opinion”, providing additional data when classifying cancers, which could help improve prognostic accuracy. It could also see use in the operating theatre – as the method employs light beams, the system could even be used on live tissue, allowing for fast analysis during surgery.
Both of these techniques require further validation before they hit the clinic, but it’s clear that the hunt for ways to make lung cancer diagnosis faster, more accurate, and less invasive for patients is yielding some very promising results.
- GA Silvestri, et al., “A bronchial genomic classifier for the diagnostic evaluation of lung cancer”, N Engl J Med, [Epub ahead of print] (2015). PMID: 25981554.
- F Großerueschkamp, et al., “Marker-free automated histopathological annotation of lung tumour subtypes by FTIR imaging”, Analyst, 140, 2114–2120 (2015). PMID: 25529256.
I have an extensive academic background in the life sciences, having studied forensic biology and human medical genetics in my time at Strathclyde and Glasgow Universities. My research, data presentation and bioinformatics skills plus my ‘wet lab’ experience have been a superb grounding for my role as an Associate Editor at Texere Publishing. The job allows me to utilize my hard-learned academic skills and experience in my current position within an exciting and contemporary publishing company.