Taking On the Challenge of Automating Mitosis Detection
Two frameworks developed to detect mitosis in color and multispectral histopathology images
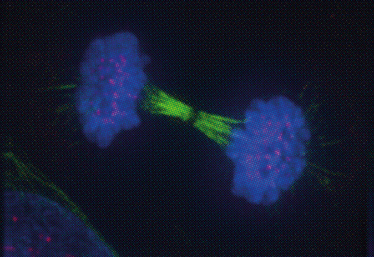
At a Glance
- Mitosis detection is an essential component of many histopathological tests, from detecting cancers to grading disease, but it’s challenging
- We propose two frameworks that detect mitotic cells in either color or multispectral images
- Automated mitosis detection may be a way of overcoming the drawbacks of manual assessment
- The future of automated mitosis detection relies on increasing the speed and sampling efficiency of digital analysis
We all know that pathological exams not only constitute the gold standard in most medical protocols, but also play a critical and a legal role in the diagnostic process. Diagnosing a disease after manually analyzing numerous biopsy slides represents labor-intensive work for pathologists. But, thanks to recent advances in digital histopathology and the ability to recognize histological tissue patterns in high volumes and definition, this laborious task has been made somewhat easier. And in my opinion, although digital pathology presents us with challenges in training, investment, standardization and computational requirements, it’s also one of the biggest evolutions in modern medicine.
Over the last decade, a huge number of articles have been published in the field of histopathology that focus on the detection, segmentation and classification of nuclei in different image modalities (1). These tasks are essential components of many histopathological applications; in breast cancer, for instance, they are involved in detection of malignancy, extraction of prognostic features, nuclear pleomorphism grading as part of a computer-aided prognostic system, detection of lymphocyte infiltration, and assessment of tumor proliferation.
Mitotic count is known to be an important parameter for disease prognosis, particularly in cancer – but actually detecting mitotic nuclei, even with digital histopathology, is a real challenge to us; they’re small objects that vary widely in shape and texture. This has never been addressed well in the literature, mainly because of the lack of available data, so in a bid to plug this research gap, an international contest, the MITOS benchmark (2), was launched at the 2012 International Conference on Pattern Recognition (ICPR). The contest challenged teams to identify all mitotic figures in a region of interest of H&E-stained tissue, using three scanners – the ScanScope XT (Aperio ePathology from Leica Biosystems), the NanoZoomer 2.0-HT (Hamamatsu Photonics), and a multispectral microscope. The mitotic nuclei had been annotated manually in each high-power-field (HPF), but the goal of our research was to develop frameworks that were able to automatically detect mitosis in breast cancer tissue on these different types of scanners.
We proposed two different frameworks: the first is Intensity, Texture and Morphology based Mitosis detection in Color images (ITM2C) (3); the second is Multispectral Intensity, Texture and Morphology based Mitosis detection in Multispectral images (MITM3) (4). I’ll explain how each works in turn.
Using color as our guide
The ITM2C framework is designed to feature a high detection rate, low incidence of false positives, and good discrimination between mitotic and non-mitotic regions. Its overall aim is to improve the accuracy of mitosis detection by integrating the color channels that best capture the statistical features of mitosis. To do this, it employs three main steps: color channel selection, candidate detection and segmentation, and feature computation and classification (3) (Figure 1).
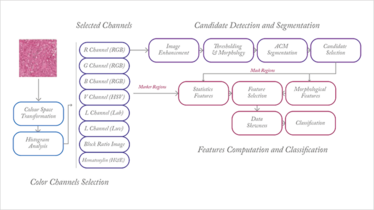
Figure 1. Mitosis detection framework (ITM2C) for color images. ACM, Active Contour Model.
Step one
Histogram analysis of different tissue components using numerous color channels, blue ratio and hematoxylin images. Histograms where the peaks of the mitotic region are different from those of other regions are selected for further analysis.
Step two
Candidate detection includes smoothing, binary thresholding and morphological operations to generate candidate regions whose boundaries are then further refined. This step is performed using the red color channel (where peaks between regions show the best separation).
Step three
Computation of the features of each candidate region in all selected color channels; a subset of features are chosen to maximize consistency.
Finally, after an extensive investigation to infer the best mitotic classifiers, the candidate region is classed as either mitotic or non-mitotic.
Multiple bands better than one?
The MITM3 framework addresses two important additional questions. First: is spatial-spectral analysis on selected spectral bands – as opposed to on a single band or all bands – satisfactory for efficient classification of mitotic and non-mitotic nuclei? An obvious advantage of using selected bands is the reduced computational and storage complexity. And second: how effective are multiple features for discrimination of mitotic and non-mitotic nuclei compared with using a single feature?
This framework is novel in several ways – it uses three different methods for spectral band selection, it computes morphological and multispectral statistical features (MMSF), and it extensively investigates classifiers and infers the best one for defining mitotic nuclei. Five steps are involved (Figure 2).
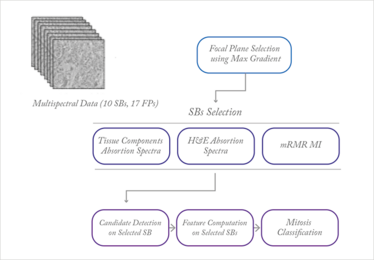
Figure 2. Mitosis detection framework (MITM3) for multispectral images. SB, spectral band; mRMR, maximum redundancy maximum relevance.
Step one
Choosing the most informative focal plane for separating mitotic nuclei from background.
Step two
Selecting relevant spectral bands for detecting mitosis. We suggest three different methods for spectral band selection: relative spectral absorption of different tissue components, spectral absorption of H&E stains, and the minimum redundancy maximum relevance (mRMR) technique.
Step three
Detection of potential mitotic nuclei.
Step four
Computation of a MMSF signature vector of intensity and texture information for each candidate across selected spectral bands. Morphological features are also computed using segmented regions of the candidates and are added to the signature vector.
Step five
Candidates are sorted into mitotic and non-mitotic classes using an L-SVM (linear support vector machine) classifier.
A side advantage to performing simultaneous analyses on multiple spectral bands is that we can investigate whether this yields an improvement in accuracy over using just one or all spectral bands. In addition, both patch- and region-based features can be evaluated for mitotic discrimination; in our case, the framework shows better classification results on patch-based, rather than region-based, texture features.
Our frameworks rank highly among the ICPR MITOS contest results, coming first overall (based on F-measure accuracy score) for multispectral and second for Aperio and Hamamatsu datasets. In addition, the frameworks perform almost equally well on brightlight and multispectral data – a promising result on the way to clinical applications.
Where next?
In the past 10 years, the digital detection, segmentation and classification of nuclei has moved to the forefront of histopathology research. Differences in slide preparation, image acquisition or complexity of tissue structure can often result in a high degree of variability when viewing routinely stained images, which in turn, makes nuclear detection – and in particular mitotic count – very challenging. The little research activity in this area means that we still have some way to go in detecting and segmenting mitotic nuclei to allow us to accurately and reproducibly use this parameter for disease prognosis. We’ve proposed two frameworks for different types of digital datasets, both of which have been carefully evaluated and have performed very successfully so far. But this isn’t the end of our work – next, we plan to expand from a two-class into a multi-class sorting system and then use it to classify all kinds of microscopic objects, from apoptotic nuclei to cancerous cells.
Frameworks like ours can improve the reliability of mitotic activity assessment, which is a key component in the histological grading of cancers. Mitotic counts are traditionally done by visual estimation through a microscope, but this technique has less than optimal reproducibility. Automated mitosis detection offers a quantitative measure to describe tumor proliferation and may be a way of overcoming the drawbacks of manual assessment. Similar frameworks can be applied to other histological characteristics, like nuclear atypia or lymphocyte infiltration, both important parameters in breast cancer diagnosis and prognostic evaluation. In my experience, any laboratory task that involves object detection and pattern recognition can benefit from automation.
And the drawbacks of these frameworks?
The main challenge to implementing computer-aided techniques like the ITM2C and MITM3 frameworks is that they are computationally expensive; at the moment, mitosis detection in a 0.5 x 0.5 mm image takes 10–20 minutes – an unacceptable amount of time considering that a typical histopathology slide contains a tissue area of about 15 x 15 mm.
In the near future, we hope that increasing the efficiency of digital analysis will improve detection speeds and result in increased use of automated methods. Because this work is so laborious, but so important to the diagnostic and prognostic process, researchers are developing new frameworks to improve the accuracy and reliability of computer-based mitosis detection. These frameworks offer an opportunity to quantify results and ensure reproducibility, but they bring with them new challenges. We need to speed up automated detection to make it a viable option for overworked laboratories, and we need to devise more efficient sampling tools for fast and accurate classification of microscopic objects. Once we have tackled these challenges, though, the future of automated histopathology is a bright one.
- H. Irshad et al., “Methods for Nuclei Detection, Segmentation and Classification in Digital Histopathology: A Review. Current Status and Future Potential”, IEEE Rev. Biomed. Eng., 7, 97–114 (2014).
- L. Roux et al., “Mitosis Detection in Breast Cancer Histological Images: An ICPR 2012 Contest”, J. Pathol. Inform., 4, 8 (2013).
- H. Irshad, “Automated Mitosis Detection in Histopathology using Morphological and Multi-channel Statistics Features”, J. Pathol. Inform., 4, 10 (2013).
- H. Irshad et al., “Multispectral Band Selection and Spatial Characterization: Application to Mitosis Detection in Breast Cancer Histopathology”, Comput. Med. Imag. Graph., 38, 390–402 (2014).
Humayun Irshad is Research Fellow at Harvard Medical School, Beth Israel Deaconess Medical Center, USA.