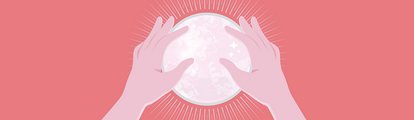
Proteomes and Predictions
How advanced spatial phenotyping can help predict tumor sensitivity and resistance to immunotherapy
Niyati Jhaveri, Arutha Kulasinghe | | 11 min read | Practical
Immunotherapies, in particular immune checkpoint inhibition (ICI), can lead to remarkable outcomes for a subset of solid malignancies. But overall response rates remain frustratingly low, with success primarily in patients with non-small cell lung carcinoma (NSCLC), renal cell carcinoma, and melanoma (1). Given these odds, predicting which patients will benefit from immunotherapy is of paramount importance. Stratifying likely responders and non-responders can offer a range of important benefits:
- guiding treatment decision
- selecting patients for clinical trials
- minimizing the risk of subjecting patients to the rigors of treatment, costs, and potentially serious adverse events if they are unlikely to respond.
- developing combination therapies for non-responders targeting the resistance, making cold tumors hot by driving the desired immune cells into the tumor.
Predictive approaches hitting their limits
An understanding of the tumor microenvironment (TME) and immune checkpoints led to the development of checkpoint inhibitors, including those that target the programmed cell death 1 (PD-1) pathway. Interaction of the PD-1 ligand (PD-L1) on tumor cells with PD-1 on T cells reduces T cell function, limiting the ability of the immune system to attack the tumor. Checkpoint inhibitors block the interaction of PD-L1 and PD-1, releasing the brakes applied to the immune system by the tumor.
Predicting patient response to ICI currently relies on PD-L1/PD-1 immunohistochemistry (IHC), next generation sequencing (NGS), and assessment of the tumor mutation burden (TMB) status (2). The US Food and Drug Administration (FDA) has granted approval to multiple drugs based on these assays as the triaging tool for patient eligibility (3). These assays, however, are limited in their predictive power because they provide bulk measurements across an entire tissue sample – measurements that are not representative of a dynamically changing TME.
A recent meta-analysis review assessed the predictive accuracy of PD-L1 IHC, TMB, gene expression profiling, and mIHC/mIF in predicting response to anti-PD-1/PD-L1 therapy (4). This meta-analysis of pre-treatment tumor specimens encompassed more than 50 published studies, 10 different solid tumor types in 8,135 patients, and correlated this with anti-PD-1/PD-L1 response (see Figure 1). Each study had assessed different types of biomarker assays for their value in predicting patient response to anti-PD-1/PD-L1 therapy.
In the review, mIHC/mIF had a significantly higher diagnostic accuracy and a higher positive predictive value (PPV) and positive likelihood ratio (LR+) when it came to identifying patients most likely to respond to therapy. The PPV and LR+ translate into fewer false positives – patients who would be treated with anti-PD-1/PD-L1 therapy, but do not respond to therapy. It should be noted that the improved performance of mIHC/mIF cannot simply be attributed to a larger number of analytes as the gene expression profiling methods assayed a median of 17 analytes versus 2--3 for mIHC/mIF. As such, spatial signatures may have played a crucial role in this scenario.
Overall, the findings suggest an improved diagnostic benefit when spatial relationships and protein co-expression on cellular subpopulations are assessed – and that measuring cell phenotype and functional states is likely to provide a real-time view of the dynamically changing TME.
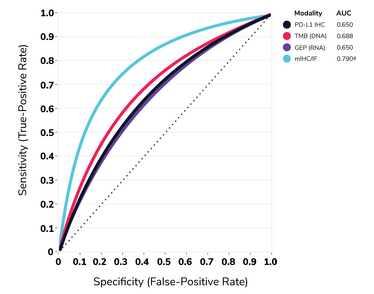
Figure 1. A meta-analysis of different diagnostic techniques for predicting response to PD-1/PD-L1 checkpoint inhibitor immunotherapy showed that multiplexed mIF/mIHC methods had higher predictive value than currently used assays, including PD-L1 IHC, TMB and, gene expression profiling. The “a” indicates statistical significance (P < 0.05), Hanley and McNeil method. Credit: Akoya
In addition, the results of several clinical trials using single biomarkers have not been consistent and some are even contradictory (5–7). Not all PD-L1+ patients respond to immunotherapy and some whose tumors score negatively using PD-L1 IHC respond to anti-PD-1 ICI (8).
Other trials have reported conflicting results when using PD-L1 as a prognostic biomarker because of the heterogeneity of antibodies used for detection or the PD-L1 positivity threshold (9). More harmonization studies are needed to elucidate optimal antibody clones, staining patterns, and associations with clinical endpoints.
Shedding light on tumor heterogeneity
Reliance on single biomarkers that use binary cutoffs yields a limited snapshot of information to guide treatment-related decisions. A single stain simply cannot provide the insights into tumor heterogeneity and TME dynamics needed to determine whether a patient is likely to respond to a particular therapy. In addition, the TME is more reflective of the dynamic nature of the tumor changing under the pressures of therapy.
By accounting for the complex nature of the TME and providing a more detailed, functional profiling of the tumor, its environment, and interaction with the immune system within a spatial context, we have the potential for more accurate prediction of patient response and outcomes.
Spatial biology, especially spatial phenotyping, is a powerful technique for quantifying protein co-expression on immune cell subpopulations and assessing their spatial arrangement within the microarchitecture of the TME (10). The technique combines ultrahigh-plex protein detection, high-throughput workflows, and powerful analysis algorithms, enabling more than 100 biomarkers to be visualized, characterized, and quantified in a single tissue sample at single-cell resolution – while preserving whole-slide spatial context.
As the morphological landscape of the TME is retained, unique geographic, temporal, and phenotypic signatures are revealed. Spatial distribution and quantification of tissue-specific proteins involved in different pathways, including immune activation, metabolism, stress, and invasion, becomes possible – along with simultaneous identification of distinct cell types and their functional states. The ability to map the spatial proteome reveals the disruption of the normal organization of immune, epithelial, stromal, vascular, and other cell types, offering the potential for a more detailed view of tumors and visually revealing the factors driving sensitivity and resistance to immunotherapy.
Unlike conventional, low-plex assays (which are limited to analyzing a handful of biomarkers per sample), mapping the spatial proteome of a tumor can uniquely answer the following important questions:
- Where and what types of cells are present in the TME?
- What are the tumor and immune cell activation status?
- What biomarkers are they co-expressing?
- How are the cells organized and interacting?
- What cell niches/neighborhoods are present?
- What subpopulations of cells are associated with clinical endpoints?
- What factors are driving sensitivity or resistance to treatment?
- What changes are occurring in response to treatment?
Establishing a foundation for personalized treatment
Several studies have used highly multiplexed spatial phenotyping of the tumor immune microenvironment to improve the stratification of patient responses to immunotherapy.
One study employed a 56-plex antibody panel to reveal the presence of distinct cellular neighborhoods (CN) in advanced colorectal cancer (CRC) tissue. The authors developed technology and an analytical framework to systematically describe the components of complex tissue types and their coordinated behaviors. They liken the tumor immune microenvironment to a city composed of distinct neighborhoods where specific functions occur. These neighborhoods are distinguished by their composition of buildings, activities, and people, but they exhibit behavior of their own (11).
The authors identified a correlation between clinical outcomes and distinct organizational patterns, including neighborhoods enriched for tertiary lymphoid structures (TLS), T cells, macrophages, and granulocytes.
In a retrospective study of cutaneous T cell lymphoma, researchers used a 55-plex antibody panel to identify differences in responsive and non-responsive cohorts following treatment with the anti-PD-1 antibody pembrolizumab (12). Neither single-plex IHC studies for CD4, PD-1, PD-L1, and FOXP3, nor an analysis of TMB revealed any significant differences between the two groups. In contrast, use of the multiplex biomarker panel revealed a higher frequency of CD4+ immune-activated CNs in responders versus non-responders, as well as significantly higher levels of Treg-enriched immune-suppressed CNs in the non-responding cohort before and after pembrolizumab treatment.
Seeing resistance for the first time
We recently authored a peer-reviewed publication that described mapping of the spatial proteome of head and neck squamous cell carcinoma (HNSCC) to visualize tumor resistance to ICI with ultrahigh-plex whole-slide imaging for the first time (13). ICIs are used to treat recurrent/metastatic HNSCC with a durable benefit observed in approximately 30 percent of patients. Current biomarkers for HNSCC, however, are limited in their ability to accurately predict responses to therapy and guide patient treatment regimens.
Our study optimized and applied an ultrahigh-plex, single-cell spatial protein analysis on formalin-fixed paraffin-embedded cancerous tonsil tissue from a patient who initially responded to ICI treatment but whose cancer ultimately progressed. The study used a panel of 101 antibodies targeting immune biomarkers as well as those related to metabolic, proliferation, invasion, apoptosis, stress, and survival pathways. By combining analysis of the spatial organization of the tumor/immune environment with functional metabolic profiling, an unprecedented visualization of patterns of treatment sensitivity and resistance was revealed.
A high degree of heterogeneity within the tumor was revealed and offered unique insights into the pattern of clinical response and subsequent progression of the cancer. Though spatial phenotyping and CN analysis based on immune and structural markers revealed multiple tumor-immune CNs within the tissue sample, analysis of metabolic and functional phenotypes revealed a more nuanced profile across all cell types contained within the sample.
The tumor mass consisted of four distinct regions based on histological examination of the tissue, expression of epithelial markers, and spatial metabolic phenotyping (see Figure 2):
- Region 1 had a high degree of apoptotic cells
- Region 2 had a central apoptotic core surrounded by a rim of proliferating cells
- Region 3 presented a more active tumor phenotype involved in tumor promotion and metastasis relative to the rest of the tumor mass
- Region 4 was highly dynamic and primed with the energy requirements for apoptosis and immune activation.
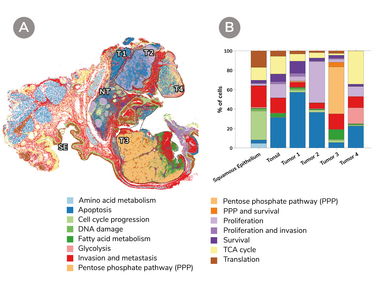
Figure 2. Whole-slide single-cell spatial phenotyping of a human FFPE head and neck squamous cell carcinoma with an ultrahigh-plex antibody panel reveals four distinct tumor regions with varying metabolic and stress profiles. A. Tumor 1 (T1) has a high degree of apoptotic cells (blue); Tumor 2 (T2) shows a central apoptotic core (blue) surrounded by proliferating cells (light purple); Tumor 3 (T3) shows high expression of markers for the pentose phosphate pathway (peach), fatty acid metabolism (green), and invasion (red); and Tumor 4 (T4) is highly dynamic with cells undergoing glycolysis (light pink), tricarboxylic acid cycle (yellow), and apoptosis (blue). B. Quantification of the 14 functional phenotypes as listed in the legend shows the predominant pathways within the normal squamous epithelium (SE), normal tonsil tissue (NT), and four tumor regions illustrating the high degree of intra-tumoral heterogeneity and competing microenvironments that may inform the partial response to ICI observed in this case (13). Credit: Akoya
The distribution of immune-related cell phenotypes within the four tumor regions was explored in greater detail and compared with a small region of normal tonsil tissue that was present in the patient sample. The normal tissue had predominantly B cells (36%), T cells (41%), and vasculature (16%), while the tumor regions had a high abundance of proliferating tumor cells (25–63%) with relatively few B cells.
Region 4 had a large B cell cluster, which displayed multiple morphological features that are indicative of TLSs. It also had high levels of CD68+/CD163- M1 macrophages (18%) and CD8+ T cells (28%) suggesting high anti-tumor immune activity in this region. In contrast, region 3 had higher levels of CD68+/CD163+ M2 tumor-associated macrophages (3%) relative to M1 macrophages (0.4%).
Spatial proximity analyses of the regions further revealed that pro-inflammatory anti-tumor M1 macrophages were associated with tumor cells in region 4 whereas tumor supporting M2 macrophages were farther away. The opposite was found in region 3 where there was a closer association of M2 macrophages with tumor cells relative to M1 macrophages. These results emphasize the importance of single-cell spatial phenotyping and analyzing cellular interactions within the context of the tissue architecture to understand the complex nuances of the TME.
An assessment of this HNSCC tissue sample using a hematoxylin and eosin (H&E) stain indicated a normal tonsil (NT) region and a large tumor. Though H&E can reveal some morphological information, it is limited in terms of the ability to identify cellular phenotypes and functions. In contrast, mapping the spatial proteome offered a far greater depth of information and a more comprehensive view of the TME and key cancer hallmarks at single-cell resolution.
Overall, the study demonstrated the presence of both immune activation-induced death and tumor progression in the same HNSCC sample. These heterogenous regions and competing microenvironments may underpin differential responses to ICI therapy observed in HNSCC and other types of cancer.
Our approach represents a significant advance in ultrahigh-plex spatial mapping of the human proteome and highlights the potential utility of this technology for identification of clinically significant biomarkers, disease stratification, and understanding the underlying basis of therapeutic responses. By screening large cohorts of patients, new spatial biomarker signatures associated with therapeutic response and resistance will be revealed (14), setting the stage for better and more accurate screening tools and companion diagnostic assays for patient stratification.
Providing complementary information to current diagnostic techniques, I believe the power and potential of multiplexing and spatial biology to be immense. Ultrahigh-plex spatial approaches will continue to bring greater clarity to the complex tumor/immune cell environment, providing information on areas of therapy sensitivity and resistance, and guiding treatment decisions. Ultimately, these assays will be most valuable when advanced into the clinical setting for deeper patient stratification and determination of effective treatments based on unique spatial signatures.
Looking toward the future, as digital spatial data are imputed onto H&E images, it becomes interpretable by pathologists, making the knowledge more accessible and actionable, and democratizing spatial information for the field. As these integrated, data-rich sets provide an improved guide for selection of therapeutic regimens on a patient-by-patient basis, we can move the needle forward for precision medicine.
- A Haslam and V Prasad, “Estimation of the percentage of US patients with cancer who are eligible for and respond to checkpoint inhibitor immunotherapy drugs,” JAMA Netw. Open. 2019;2:e192535. Doi: 10.1001/jamanetworkopen.2019.2535.
- DF Hayes DF et al, “Proceedings from the ASCO/College of American Pathologists immune checkpoint predictive biomarker summit,” JCO Precis Oncol. 2022 Nov:6:e2200454. https://doi.org/10.1200/PO.22.004544.
- JD Twomey and B Zhang, “Cancer immunotherapy update: FDA-approved checkpoint inhibitors and companion diagnostics,’ AAPS J, 23, 39 (2021). doi: 10.1208/s12248-021-00574-0. PMID: 33677681
- S Lu et al, “Comparison of biomarker modalities for predicting response to PD-1/PD-L1 checkpoint blockade: A systematic review and meta-analysis,” JAMA Oncol, 1, 5, 1195 (2019). doi: 10.1001/jamaoncol.2019.1549.
- M Di Bartolomeo et al, “Prognostic and predictive value of microsatellite instability, inflammatory reaction and pd‐l1 in gastric cancer patients treated with either adjuvant 5‐FU/LV or sequential FOLFIRI followed by cisplatin and docetaxel: A translational analysis from the ITACA‐S trial,” Oncologist 2020; 25(3):e460–e468.
- JY Kim et al, “Differences in immune contextures among different molecular subtypes of gastric cancer and their prognostic impact,” Gastric Cancer, 22, 1164 (2019).
- Z Ji et al, “Hyperprogression after immunotherapy in patients with malignant tumors of digestive system,” BMC Cancer, 19, 705 (2019).
- S Wang et al, “Cancer immunotherapies: From efficacy to resistance mechanisms – not only checkpoint matters,” Front. Immunol. 12:690112. doi: 10.3389/fimmu.2021.690112
- M Nishino et al, “Monitoring immune-checkpoint blockade: Response evaluation and biomarker development,” Nat Rev Clin Oncol, 14, 655 (2017). 10.1038/nrclinonc.2017.88
- A Kulasinghe et al, “The seductive allure of spatial biology; accelerating new discoveries in the life sciences,” Immunology and Cell Biology, 101, 798 (2023). doi: 10.1111/imcb.12669
- CM Schürch et al, “Coordinated cellular neighborhoods orchestrate antitumoral immunity at the colorectal cancer invasive front,” Cell, 182, 1341-1359.e19 (2020). doi: 10.1016/j.cell.2020.07.005.
- D Phillips et al, “Immune cell topography predicts response to PD-1 blockade in cutaneous T cell lymphoma,” Nat Commun 12, 6726 (2021); doi: 10.1038/s41467-021-26974-6
- N Jhaveri et al, “Mapping the spatial proteome of head and neck tumors: Key immune mediators and metabolic determinants in the tumor microenvironment,” GEN Biotechnology, 2, 418 (2023). doi: 10.1089/genbio.2023.0029
- J Monkman et al, “Spatial insights into immunotherapy response in non-small cell lung cancer (NSCLC) by multiplexed tissue imaging,” J Transl Med 22, 239 (2024)
Discovery Applications Manager, Akoya Biosciences
Clinical-oMx Lab Head, Frazer Institute, Faculty of Medicine, The University of Queensland, Australia