Now Streaming: Spectral Simplification
A preference algorithm originally developed for Netflix could speed up Raman imaging, readying the tool for potential clinical use
Raman imaging has already shown promise as a diagnostic tool, both to identify cancer cells in tissue samples and to detect disease biomarkers. But slow imaging and the production of unwieldy amounts of data have thus far hampered its use in clinical settings, where speed and efficiency are essential. In an effort to solve the problem, a team of researchers at École Normale Supérieure in Paris has turned to a most unlikely source: Netflix.
Repurposing an algorithm originally developed in 2009 as part of a competition to develop more accurate movie preference software for the streaming giant (1), the group hoped to make use of the substantial predictive power to “fill in the gaps” in spectroscopic images. By predicting the make-up of unimaged sections of samples, the analysis time and volume of spectral data necessary to determine a samples chemical composition can be reduced.
“We combined compressive imaging with fast computer algorithms that provide the kind of images clinicians use to diagnose patients, but rapidly and without laborious manual postprocessing,” said team leader Hilton de Aguiar (2).
The team also tackled cost by replacing the camera normally associated with Raman imaging with a spatial light modulator. “The device we used is orders of magnitude less expensive and faster than other options on the market,” says Aguiar. To test the camera’s ability to distinguish high levels of chemical complexity, the team prepared samples of brain tissue and single cells, and were rewarded with their newfound ability to acquire spectral data – compressed by 64 times – in tens of seconds as opposed to the minutes or hours taken by other approaches (3).
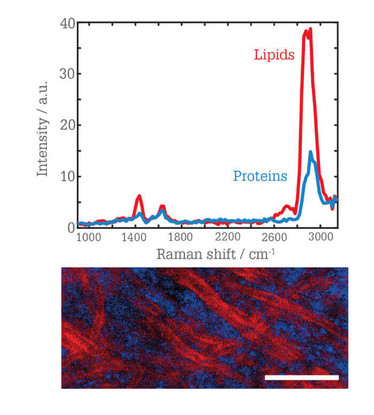
The researchers demonstrated their new methodology by using a Raman microscope to obtain spectroscopy images from opaque brain tissue. Scale bar: 20 microns. Credit: Hilton De Aguiar, École Normale Supérieure
If further testing on other biological samples proves successful, clinicians may one day gain access to a rapid new diagnostic tool, which – much to the relief of patients everywhere – will presumably not require a monthly subscription.
As an assistant editor for The Translational Scientist, I can combine two of my passions; translational science research and science communication. Having thrown myself into various editing and other science communication gigs whilst at University I came to realise the importance of good quality content that delivers in an exciting and engaging way.